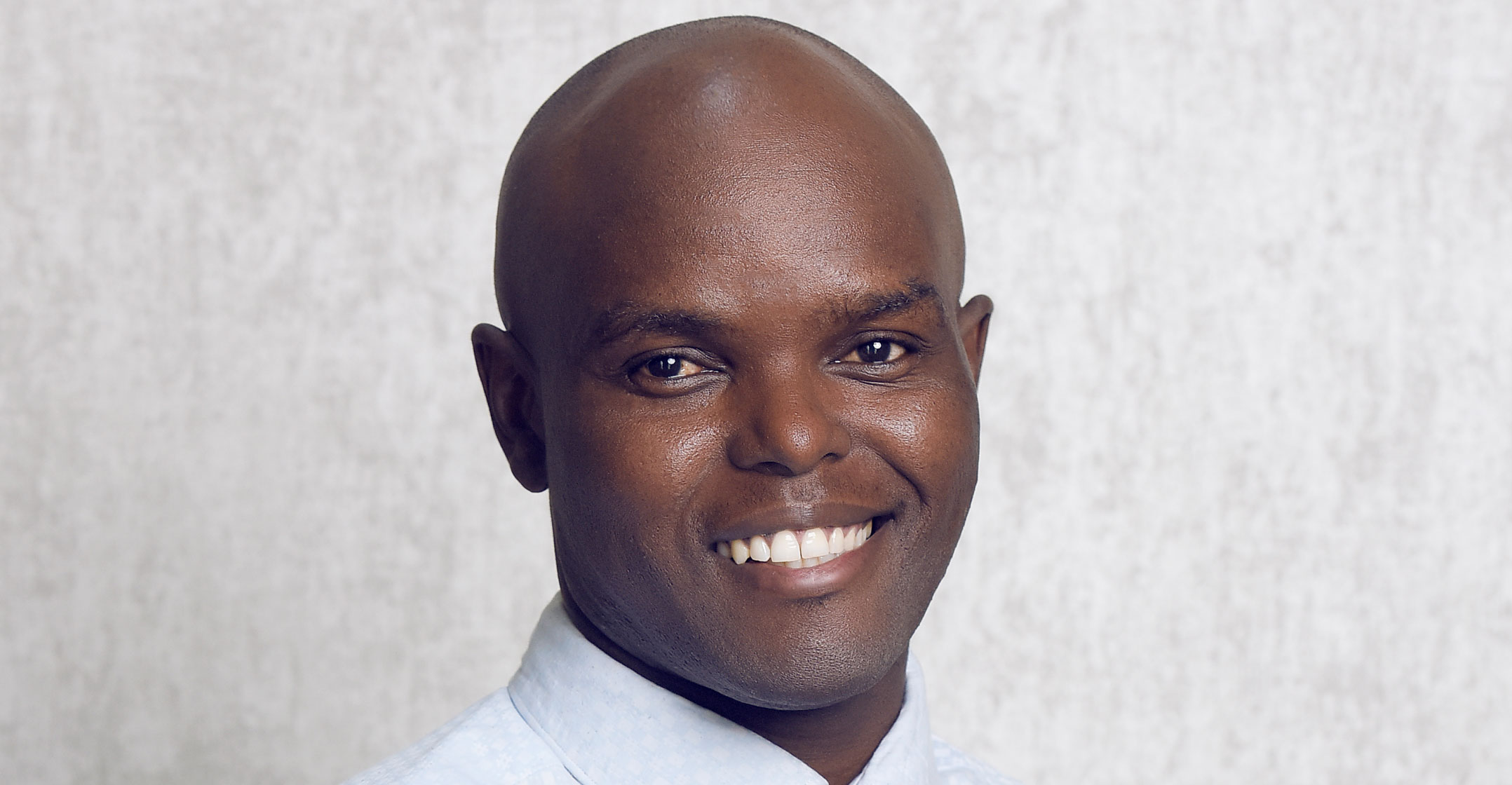
Human problem solving is finding it challenging to resolve South Africa’s complex energy crisis and artificial intelligence may be able to help.
Energy crises aren’t new, but there are new ways to respond to them. The old solutions evidently aren’t working.
Part of the problem is the sheer scale of the challenge. Myriad variables combined with legacy issues and other historical burdens that come with old energy systems and the growing demand on the grid mean that a human approach and blanket suggestions simply to build more infrastructure aren’t sufficient. The problems are too complex for the current human approach. AI, however, is adept at contending with complexity at scale.
Energy crises aren’t new. In the 1970s, just such a crisis gripped the global economy. A crude oil shortage resulted in fuel shortages in the US and Europe, affecting international import and export markets, and driving cultural changes — like a move to smaller, more efficient Japanese cars in the US and away from large, fuel-heavy local options, and the implementation of daylight savings.
Meanwhile, in the UK, because the same oil was required for heating, the government asked citizens to keep their usage to a minimum by only heating essential rooms.
Alternatives
South Africa’s energy crisis is decades old, but its effects came to the fore in 2007. Demand for electricity continued to increase, while the infrastructure underpinning it continued to degrade. Just as the US and the UK had to resort to alternatives, South Africa must expand its thinking beyond merely increasing generation capacity.
The energy crisis has affected every South African household and business, as well as cross border trade and international investment. Manufacturing has been particularly hard hit, in particular the textiles, clothing and fast-moving consumer goods sectors. There’s a domino effect at play, too. Manufacturing woes negatively impact investor confidence, both locally and internationally. South African manufacturers are wary of expanding; foreigners are wary of investing.
At the same time, intermittent power poses enormous risks for the healthcare sector, where ventilators and other critical hardware demand constant power. For consumers, beyond the inconvenience of unreliable electricity, power surges damage appliances, leading to greater spending both on the insurance and replacement of goods.
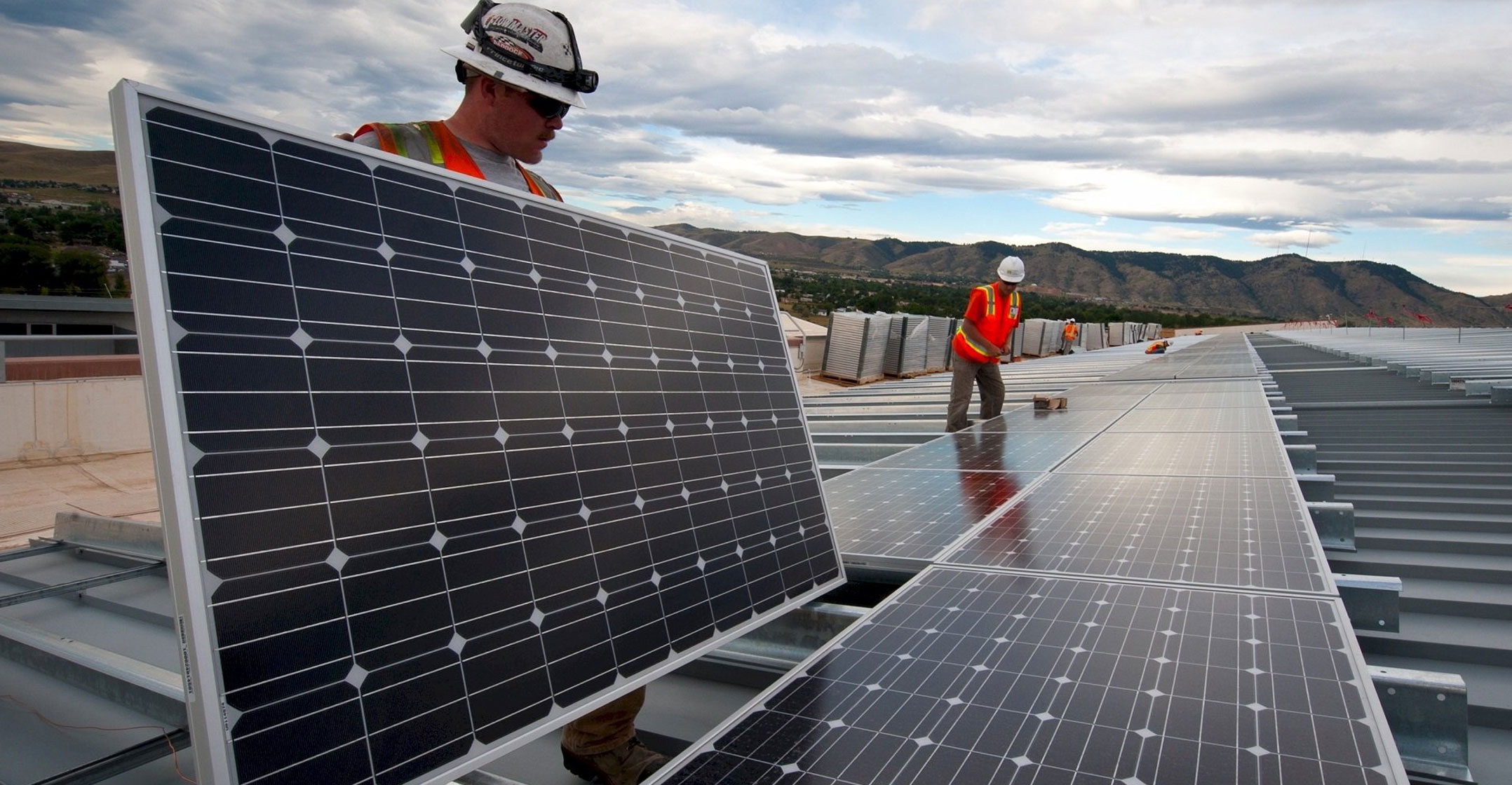
Education, which is crucial to the country’s long-term economic success, has also been impacted. Exams have been delayed or cancelled; students have found themselves needing to study at night but being left without the tools to do so.
In 2019, the CSIR estimated that between R59-billion and R118-billion was lost to load shedding, with each day of power interruptions costing the economy R1-billion. On the back of this, the World Bank cut its growth forecast for South Africa to below 1%, and the South African Reserve Bank put the estimated job losses as a direct result of the energy crisis north of 100 000.
Over the last decade, it’s estimated that the South African economy has lost R338-billion — the GDP of a small country, and devastating for one battling to recover from half a decade of near-stagnant growth and recessions.
Predictions suggest that load shedding is going to increase every year as demand climbs but the problems that led to load-shedding in the first place continue to compound.
To ensure consistent, reliable and sustainable energy supply, it’s essential that government introduces “smart” technology into the grid. Not only will this allow predictions about usage, but also for alternative energy sources to be fed into the grid.
For green energy like wind and solar to flourish, government must promote its use in the private and public sectors, provide financial assistance and subsidies or other incentives to drive its uptake, and create an investment environment that allows citizens or organisations to band together on green energy projects. Smarter grids can help produce the models to drive adoption and assist with the maintenance or expansion thereafter.
A smarter grid
Moreover, from using big data to predict usage, to using smart meters at individual premises to manage usage and minimise waste, a smart grid provides the data and facilities that AI can harness.
AI makes it possible for machines and systems to learn from experiences, adjust to new inputs, and perform human-like tasks. That is why it — and other emerging technologies — are especially well suited to help mitigate and overcome the challenges that the ongoing energy crisis poses. AI can also take the data gleaned from a smart energy sector and apply it to other industries, resulting in benefits for each, via the “fourth sector model” that combines the features of public, private and non-profit organisations.
In mature markets, power grids are becoming increasingly digitised and decentralised. Decentralisation takes place as a result of a growing number of energy production sources and business models. For that model to work, it’s critical to balance generation, distribution and demand. And that’s the role digitisation plays.
A smart grid does more than simply track energy usage, it enables the efficient transmission of energy and allows for the integration of renewable energy sources, like solar and wind. A smart grid doesn’t just manage the energy load in real time, it’s able to predict it and helps with long-term infrastructure planning. It makes the grid decentralised and digital, accommodating multiple sources.
The generation of data from a smart, connected grid is an end in itself, not merely a by-product. Planners need data to understand the grid, and sensors in it help provide this information, both immediate and historic. As the body of information grows, so do the inferences and predictions that can be made.
The result is what you might think of as an “internet of energy”. This is especially useful when it comes to unpredictable sources like solar and wind. When these renewable sources are working, the grid can harness that supply. But equally important is the ability to store it for the inevitable overcast or windless days.
To this end, Siemens has created software for smart grids — which it calls active network management (ANM) — that monitors grid inputs and outputs and autonomously makes adjustments where it can manage loads, adjust distribution to meet demand, and manage new additions to the network, all with minimal manual interaction.
Energy production and distribution is expensive but failing to do so smartly and efficiently is even more so.
Because the US has three separate grids, the US department of energy has built the creation of a smart grid into its mandate and policies. It has invested over US$4.5-billion in smart grid infrastructure (including the installation of over 15 million smart meters) since 2010.
‘Super nodes’
This investment will eventually allow for granular control of the US’s multiple grids, while also ensuring it’s ready to accommodate the 25% expected increase in demand by 2050.
Meanwhile, companies like Greensmith — which specialises in large-scale battery technology — are building intelligent energy storage infrastructure. Greensmith’s solution isn’t merely single-site storage, but distributed storage. Its batteries are organised like the nodes of a peer-to-peer network, with a number of “super nodes” issuing instructions about where to direct energy, and from which sources. That means redundancy, even if a node or control point goes down. It’s also hardware agnostic and can support multiple kinds of batteries and diverse energy sources.
These sorts of intelligent energy storage are also essential as we move away from coal and other emissions-generating production methods to renewable — but unpredictable — resources. Stem, a California company, uses AI to help map energy usage and plan storage. Another, Ready-One, uses batteries and inverters to manage peak usage and reduce buildings’ demand on the grid during peak consumption times by harnessing renewable sources instead.
To solve South Africa’s energy crisis, it’s key to have granular data, right down to the household level. That level of detail allows for equally comprehensive and detailed resource management, and the ability to both anticipate energy needs and channel energy to different parts of the grid pre-emptively, rather than reactively. Those predictions are done by machine-learning models that improve with every passing decision and as the dataset continues to grow.
Gathering this data requires dedicated hardware, but much of it can be retrofitted to existing homes or buildings. Verdigris Technologies, for example, is a California-based company that makes devices that can be directly attached to existing electrical circuits and can provide detailed insights on individual appliances, groups of devices or individual rooms’ electricity usage. This makes it possible to identify high-draw or faulty items in addition to creating valuable consumption data.
Google’s DeepMind is a great example of what’s possible when data and machine learning meet. DeepMind was able to reduce the energy required to cool data centres, which are the backbone of Google’s business. Reducing consumption didn’t merely save Google money, it also cut its environmental impact.
One of the less obvious challenges to solving the energy crisis is getting consumers behind the process. Digital engagement can help. Smart devices like Google Nest speakers, Amazon Alexa devices, and those that support Apple’s HomeKit and Siri allow end users to take control of their homes, but they can also proactively encourage better behaviour.
Connect smart assistants to tools like PowerScout — which models potential savings and uses AI as a marketplace advisor and educator — or Verv (an AI-powered home assistant that helps with energy management) and you have powerful tools for consumer education. As the grid gets smarter, these tools can also alert service providers to problems as they happen.
Eco-Bot
Then there’s Eco-Bot, a chatbot designed to help facility managers and other people responsible for managing energy usage in factories, warehouses, office parks or the like to understand energy usage down the individual appliance level. Eco-Bot’s ability to see both detailed usage and the bigger picture enables it to make recommendations in plain language, create and manage consumption targets, or identify problem areas.
In 2009, the warning system at a R16-billion Eskom power plant failed. The failure cost more than an AI system that could have predicted it and could have enabled Eskom to shut systems down before they reached a critical point.
There are many advantages to having foresight, but they’re especially acutely felt when it comes to maintenance. AI predictive models can dispatch repair crews proactively while also providing more accurate estimates for outage and repair times. Though there are initial outlay costs, the savings from avoiding downtime pay for the cost in short order.
Though some critics argue that prediction systems are inaccurate, that’s a myth. Solutions like iPredict boast an accuracy rate for predictions of more than 80%. Installing iPredict is cheaper than a massive system outage and can assist with identifying other problems on the grid, like those that stem from energy theft.
AI can also be used in conjunction with other emerging technology, like drones. The National Grid in the UK uses drones to check pylons and other infrastructure for damage or other fault detection because they can cover huge areas, handle difficult terrain, and can use infrared cameras to identify problems more efficiently than manual examination can. Add AI’s smarts, and it’s easier to create maintenance schedules and predictive diagnostics that reflect real-world conditions.
Illegal connections
Damage to infrastructure and illegal connections cost Eskom R71-million in the 2019/2020 financial year. Electricity theft, meanwhile, costs the utility approximately R20-billion/year. And though vandalism is vexing and can cause power outages, it’s not just the cost that’s problematic — damaged sites are a danger to people and animals.
AI could be used to spot discrepancies in usage patterns to identify informal connections, and machine-learning algorithms would continue to get better at identifying not just anomalies, but what is likely causing them. Systems could be designed to recognise anomalies related to damage separately from those related to theft and dispatch the correct teams accordingly.
New business models
You cannot drive digitisation without data, and without data you can’t update policies to encourage or benefit from new business models. AI will transform data into policy direction for renewable energy. It will help shape policies around everything from infrastructure investment to pricing and emissions allowances. It’ll enable Eskom to safely incorporate renewable energy while incentivising consumers to invest in alternative energy sources because their surplus production can be smartly fed into the grid for credits.
New microgrids that see community-based initiatives to share solar panels, storage devices and other energy solutions could be encouraged, especially in areas where the grid is aged, under strain or not cost effective.
The fourth sector
Data from the smart grid can be used as a gateway into helping financial organisations to drive strategies that support funding of renewable strategies. The same data can also help financial institutions understand the risk of getting into the renewable industry. Without that data, they are investing blindly. But with it, they know where and how to invest.
Moreover, public, private and non-profit enterprises can collectively benefit from the data and use it to address the ways in which the energy crisis affects them and their constituents.
Conventional solutions have failed to address the South African energy crisis. While AI and smart grids may seem outlandish now, particularly in the face of such an enormous challenge, soon they will be the global norm. By embracing them early, South Africa has the chance to position itself for energy stability in years to come while also getting to grips with a crisis that will only worsen without innovative solutions and the vision to enact them.
- Dr Mark Nasila is chief analytics officer in FNB’s chief risk office