In 1950, Englishman and renowned World War 2 codebreaker Alan Turing spelt out his test for what we now call artificial intelligence. He wrote to the effect that if a machine, irrespective of the method used, could exhibit intelligence like a human, then it should be labelled intelligent. This would become known as the Turing test.
For decades, computer scientists tried in vain to design machines that would pass the Turing test. These so-called classical algorithms, however, were not very useful in other, less-rigid fields filled with ambiguity, like languages. Unsurprisingly, AI experienced a series of winters where limited progress was made – from 1974-1980 and then from 1987-1994.
In 1958, Frank Rosenblatt, from Cornell Aeronautical Laboratory, devised a novel approach. Using a giant computer, he demonstrated the “perceptron”, a simple artificial neural network. This was inspired by how the human brain was thought to work with neurons (nodes) and synapses (numerical weights). But artificial neural networks did not take off as there was not enough computing power.
After the second AI winter, AI hit the limelight in 1997 when world chess champion Gary Kasparov was defeated by IBM’s Deep Blue computer. But this was still not the AI of today. Deep Blue “relied mainly on a programmed understanding of chess”.
Geoffrey Hinton is our second brilliant Englishman. Based in Canada, Hinton started working on neural networks in the late 1970s and the early 1980s, when the field was largely left for dead. He said the “whole idea was to have a learning device that learns like the brain… Turing had the same idea and thought that was the best route to intelligence.” Hinton co-wrote a paper on “back-propagating errors”, popularising the concept of artificial neural networks.
In 2012, PhD student Alex Krizhevsky, in collaboration with others, including Hinton, entered the ImageNet Challenge. The dataset consisted of over a million images, and the challenge was “to evaluate algorithms designed for large-scale object detection and image classification”. They used an artificial neural network that Krizhevsky designed, and two GPUs (graphics processing units) made by Nvidia. AlexNet, as it was named, won the challenge, and it became clear that deep learning using large neural networks was the way forward for AI.
Feels like 1999
Nvidia was then known as a provider of 3D graphics chips for computer games. These chips, or GPUs, were designed for specific, repetitive tasks like accelerating the rendering of images on a screen. GPUs are like a truck – slow but can carry a lot – while CPUs are like a Ferrari but cannot carry much. But before the GPUs of old would be of any use in an AI challenge like ImageNet, they needed to be adapted for broader use. Nvidia introduced Cuda, a new programming language, in 2006. Cuda allowed GPUs to be reprogrammed for other purposes.
GPUs are vital in the first leg of deep neural networks – training – where a computer sifts through large datasets. It mimics how a brain works and eventually draws accurate conclusions. Nvidia dominates this market. Once the model has been trained, it is ready for inference where, via a chatbot, the model can predict the specific answer. Training is like getting an education, while inference is like applying that education in a job.
OpenAI is where we close our history of AI. In late 2015, Sam Altman, Elon Musk and a team of top AI researchers unveiled a new AI business, OpenAI. Since then, OpenAI has captured our imagination by releasing generative AI products like ChatGPT and Dall-E.
Microsoft first invested in OpenAI in 2019, an investment driven by Satya Nadella, the CEO. With a 49% stake in OpenAI, Microsoft is now regarded as a generative AI leader and is using this caché to draw more customers to its cloud computing division.
Meanwhile, interest in other AI start-ups has soared, and spending on computing power required for running large language models is rocketing. Nvidia is the new Cisco. It feels like 1999!
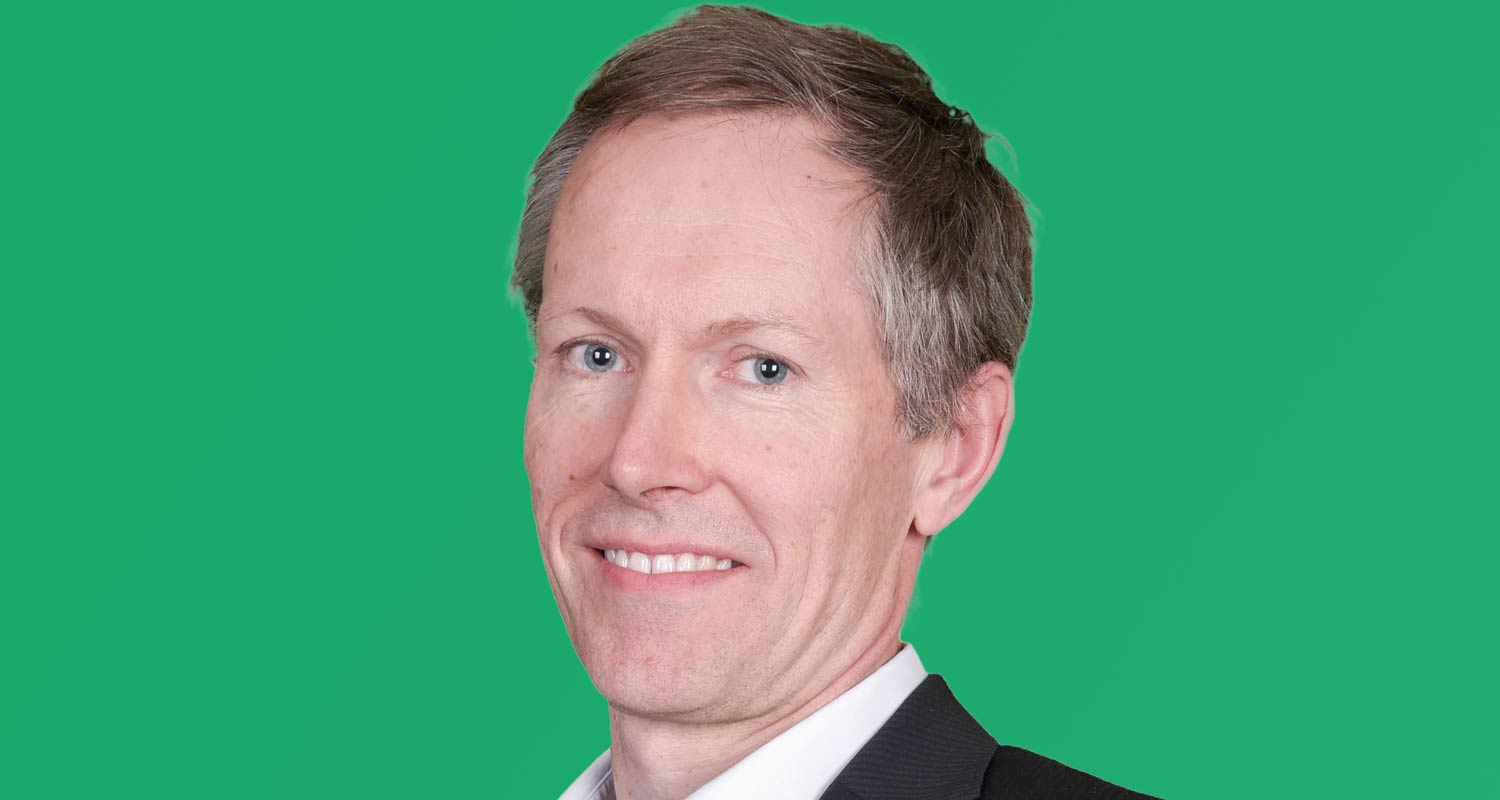
Where has modern AI come from, and where is it going? The first major development in modern AI was image recognition. This was the era of recognition, but we have now moved into the era of generation. LLMs will improve and become more efficient at training. Different specialisations are developing as LLMs move into health care, finance, law and so on. Experts predict reasoning will follow generation. LLMs do not currently do chains of reasoning very well.
The AI endgame appears to be achieving artificial general intelligence (AGI), a step up from generative AI. We are not there yet. Ian Hogarth recently wrote an essay in the Financial Times, warning of the God-like qualities of AGI – which “understands its environment without the need for supervision and … can transform the world around it”.
With the move out of academia into industry, the AI genie is out of the bottle. To paraphrase Henry Kissinger et al, it will take a global effort to define our relationship with AI and the resulting reality.
Ray Kurzweil, a respected AI futurist, believes AI will finally pass the Turing test in 2029. Humans can then connect their neo-cortex, the section of the brain where we do our thinking, to AI via the cloud. There are some rudimentary moves in this direction, with companies like Neuralink. Kurzweil expects this to happen in the 2030s. In other words, humans will merge with AI in the next decade.
Suppose AI is as disruptive to the global economy as other momentous new technologies; we should expect the usual short-term pain for long-term gain. Specific jobs may become redundant while new types of jobs are created. After the initial disturbance, productivity growth typically accelerates, bringing long-term benefits. It may be too early to say if AI will be any different.
- David Gibb is a fund manager at Anchor Capital, an international, independently owned boutique wealth and asset management firm with some R110-billion in assets under management and advice
- Read more articles by David Gibb on TechCentral