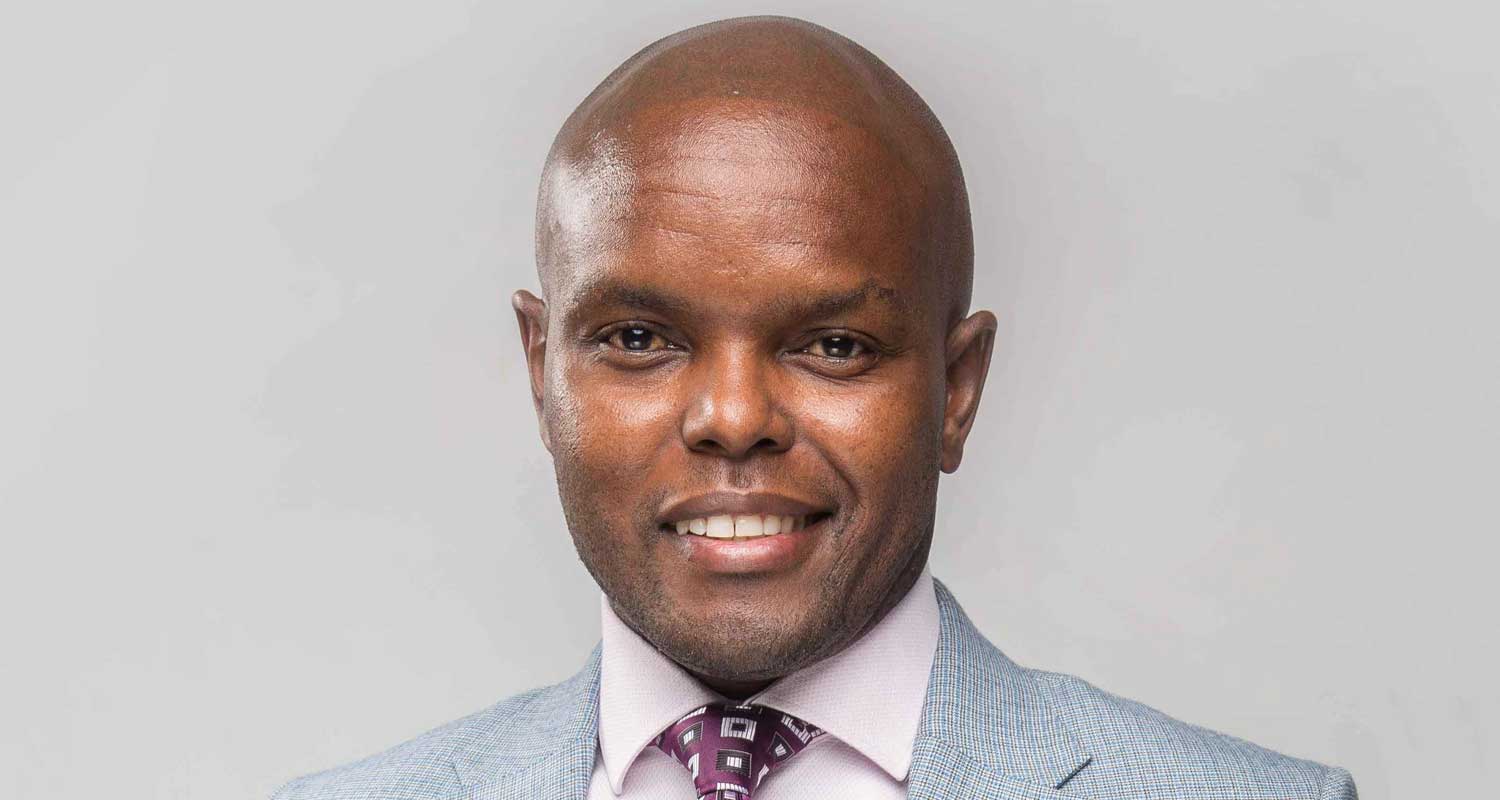
Artificial intelligence infrastructure refers to platforms allowing the development of intelligent applications that are predictive, self-healing and require minimal human interaction. It plays a crucial role in the success of enterprises undergoing AI-driven transformations. The changes brought by AI to workloads and operational goals necessitate modifications in how raw data is managed, even at the physical layer of the data stack.
AI is already influencing the design of infrastructure, including edge computing. In addition to processors, hardware is being optimised to support AI workloads. However, configuring hardware to effectively handle AI requires coordinated effort and flexible approaches. There is no one-size-fits-all solution for implementing AI infrastructure.
Aligning AI technology with business strategy
Technology alignment and AI business strategy play a vital role in the success of organisations in an increasingly competitive global market. Ensuring that technology choices and investments are aligned with strategic objectives is essential. This process involves harmonising the organisation’s vision, culture, processes and resources with the technological needs and opportunities of the market.
There’s also the issue of “technical debt” to consider. In software development, technical debt refers to the cost incurred when choosing an easier but less optimal solution, leading to additional rework in the future, and with it, additional cost.
A survey conducted by McKinsey with CIOs from financial services companies revealed that a significant portion (10-20%) of the technology budget allocated for new products is redirected to resolving issues related to technical debt. Moreover, CIOs estimated that technical debt accounts for 20-40% of the value of their entire technology assets before depreciation.
What is AI washing?
AI washing refers to a marketing practice where vendors falsely claim that their products or services incorporate AI technology, even when the connection to AI is minimal or non-existent. It is akin to “greenwashing”, where companies make misleading or unsupported claims about the sustainability of their offerings.
A study by London-based company MMC Ventures looked at 2 830 startups across Europe and found that 40% of those claiming to be “AI startups” had little to no AI capabilities at all. To avoid falling prey to AI washing, it can help to consider the following:
- Does the vendor actually have AI experience and the resources required for AI, like data scientists, data architects and data engineers?
- Does the vendor have significant data for its AI applications and does this data come from a variety of sources?
- Can the vendor demonstrate it has the appropriate AI infrastructure, from data centres to DevOps teams?
- And finally, do the vendor’s AI capabilities align with the objectives an organisation is trying to solve?
Further questions to ask
As innovation and technology advance, it is important to consider the following questions when making decisions: 1. Does this technology align with our mission and support our strategic direction? 2. Will this new technology integrate with our existing systems? 3. How much of it do we really need? 4. Does this technology fit in our budget?
By addressing these questions, organisations can make informed decisions about adopting new technologies that align with their mission, integrate with existing systems, meet their needs and provide a satisfactory ROI within their budget constraints.
The value of AI infrastructure
Intelligent infrastructure is crucial for harnessing the full potential of AI systems, especially as businesses recognise the value of integrating AI into their operations. AI infrastructure supports every stage of the machine learning workflow. By automating processes and leveraging AI, businesses can enhance operational efficiency, reduce costs and transform customer service, for instance through AI-driven chatbots.
Globally, the AI infrastructure market is expected to reach $122.8-billion by 2028, according to reportlinker.com, at a market growth of 24.7% (compounded annually). A survey conducted by IDC revealed that business leaders increasingly recognise the importance of purpose-built infrastructure for AI to deliver meaningful value to their organisations.
Insufficient infrastructure was identified as a major factor behind unsuccessful AI projects, hindering progress in over two-thirds of surveyed organisations. This highlights the crucial role of appropriate infrastructure in the success of AI initiatives.
When determining the infrastructure solution for AI platforms, enterprises should have a clear understanding of the data life cycle from the perspective of AI models and the specific requirements of these models.
Here are some important considerations relating to data that organisations need to know before defining AI infrastructure: Efficient management and storage of unstructured and semi-structured data; challenges in gathering data from multiple sources; complex processing for meaningful insights; high-performance computing platforms and engines; high-performance computations for deep neural network models; and efficient storage and throughput.
Core components of AI infrastructure and technology requirements include well-organised data management and a data architecture for collection, storage, transformation, distribution and consumption. Ingestion involves collecting and labelling raw data for AI models, which can come from static datasets or ever-changing sources.
Data storage must handle massive amounts of structured and unstructured data in a scalable data lake. Data preparation involves cleansing and transforming data in the data lake for AI model training. Data access ensures reliable access to structured data stores for AI systems.
The next steps include feeding processed datasets to AI/ML tools, training and evaluating models with high-performance storage, and using different ML models for specialised tasks. After training, AI models are used for scoring or prediction in production environments. Redundant data is stored in low-cost storage. Finally, inference occurs when the AI takes action based on its analysis of incoming information.
It is crucial to align business objectives with the implementation of AI and related efforts. By doing so, companies can ensure that the chosen technologies support their overall goals and facilitate a smoother transition.
- The author, Prof Mark Nasila, is chief data and analytics officer in FNB’s chief risk office
- Read more articles by Mark Nasila on TechCentral
- This promoted content was paid for by the company concerned