Just as the public health advances of the 19th century were driven largely by investments in abundant, clean water, the digital health advances of the 21st will be propelled by abundant, clean and healthy data. The parallel is clear: where clean water once eradicated diseases and improved life expectancy, healthy data now promises to revolutionise healthcare, driving efficiency, precision and better patient outcomes.
As we move further into the 21st century, the importance of healthy data cannot be overstated. Just as clean water was the cornerstone of public health in the past, clean data will be the foundation of digital health in the future. By ensuring that the data feeding into AI systems is accurate, complete and free from bias, we can unlock the full potential of AI to transform healthcare, making it more efficient, effective and equitable for all.
Starting with the killer app
When embarking on the AI journey in healthcare, the consensus among experts is clear: start small and focused. As Henry Adams, country manager for InterSystems South Africa, explains: “You have to find a clear use case, the killer app – something manageable yet significant enough to demonstrate the value of AI quickly.”
This approach resonates with the experiences of healthcare systems worldwide. For instance, in the Middle East, specific AI use cases, such as predicting clinic no-shows or managing chronic diseases like diabetes have been implemented with varying degrees of success. The key takeaway? Focus on a targeted project where AI can deliver immediate and tangible benefits. This not only proves the concept but also builds confidence among stakeholders.
The importance of healthy data
Before diving into AI, healthcare organisations must first focus on their data. According to Adams, getting the data ready is a journey – it’s about creating healthy data, which is foundational for any AI application. This is not just about data collection but ensuring that the data is accurate, structured and comprehensive, with appropriate governance.
Dr Hervé Rivière, physician executive, InterSystems, says: “Data is essential for effective patient management, and structured data allows for better patient management, reducing the risk of medical errors and improving the overall quality of care. This is particularly critical in settings where multiple clinicians interact with the same patient, requiring a seamless and accurate exchange of information across the care continuum.”
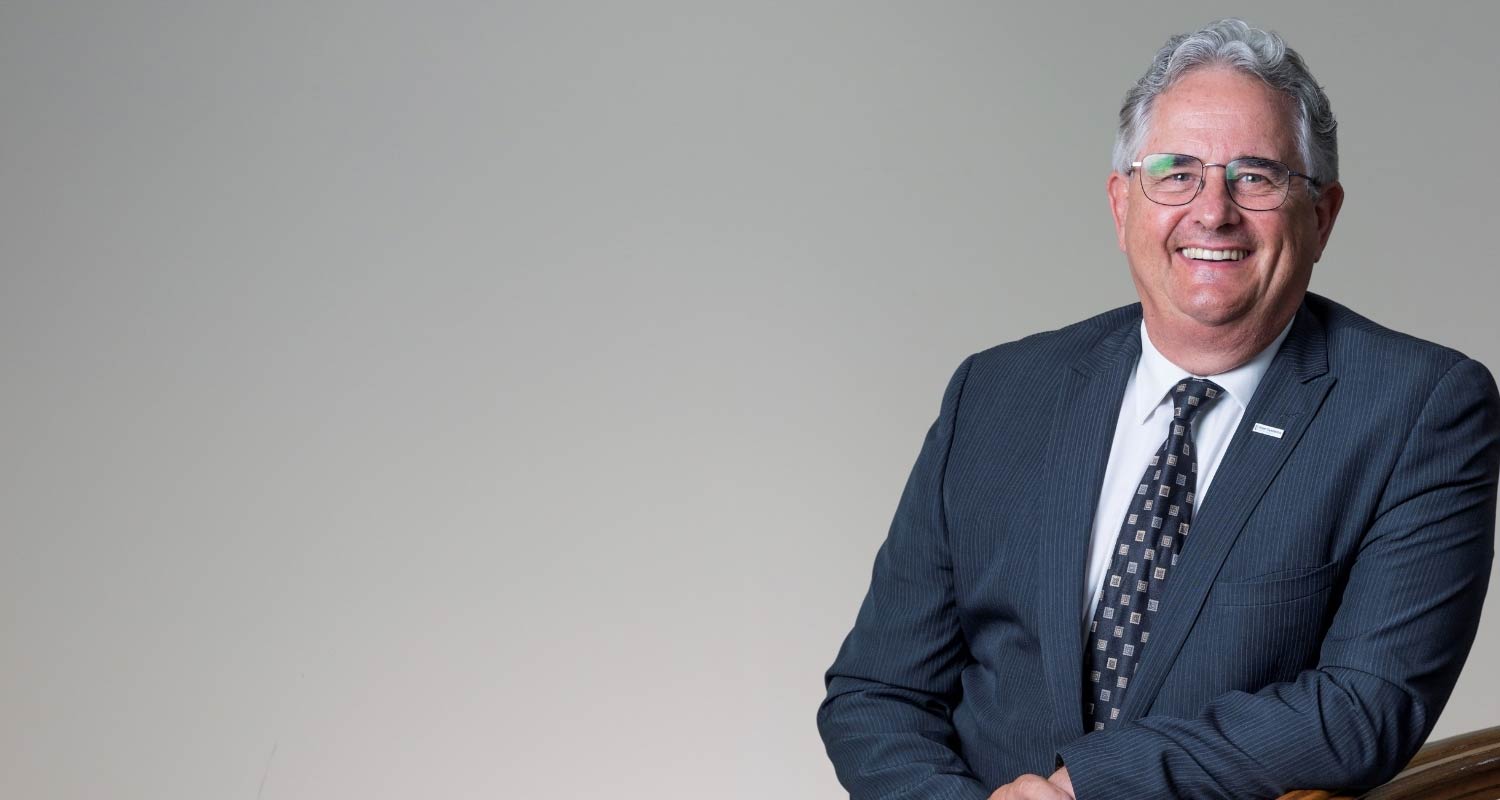
But it’s not just clear sailing. One of the significant challenges in deploying AI in healthcare is navigating the regulatory landscape. Privacy, consent, transparency and data security are paramount concerns, especially in regions with stringent regulations like GDPR in Europe or Popia in South Africa. As well as countries enforcing their data sovereignty and safety with their specific AI laws such as the EU’s landmark AI Act, which went into effect on 1 August 2024.
Preparing for failure and learning from it
As with any innovation, AI in healthcare comes with risks. “One of the pieces of sage advice is to be prepared to fail,” says Adams. The hype around AI can lead to unrealistic expectations, and it’s important to acknowledge that not every project will be a success. This is particularly true in the pilot stages of adoption where the technology is still maturing and regulatory frameworks are being developed. Industry analyst firm Gartner predicts that 80% of AI projects will underperform, and at least 30% of generative AI projects will be abandoned after the proof-of-concept stage.
However, failure should not be viewed negatively. Instead, it should be seen as part of the learning process, helping organisations refine their approach and eventually achieve success. The advice here is to start with smaller projects where the risks are manageable but the potential impact is significant. This allows for a more agile approach, with lessons learned quickly applied to future initiatives.
The role of AI in reducing costs and improving care
One of the most compelling reasons for the future adoption of AI in healthcare is its potential to reduce costs while improving the quality of care. Rivière points out: “By increasing the quality of care, AI can also reduce costs. For example, AI should be able to play a part in helping to predict and prevent complications in chronic disease management, thereby reducing hospital readmissions and the overall burden on healthcare systems.”
There could also be a role for AI in administrative functions, such as revenue cycle management (RCM), which is equally important. In regions like South Africa, where accurate billing and billing codes are critical, AI may be able to help capture all billable services with sufficient context to help reduce the risk of revenue loss. This is especially relevant in private hospital settings, where complex procedures and treatments require meticulous documentation and coding, and freeing doctors to spend more time with patients and their care should aid productivity, experiences, and outcomes.
Building a trusted partnership
The road to AI in healthcare is long and complex, but the journey begins with a clear focus on data. By starting small, preparing for challenges, being willing to fail and building trusted partnerships, healthcare organisations can unlock the full future potential of AI.
“We are at the beginning of the AI story in healthcare. It’s a journey that requires responsibility, caution, and a deep commitment to improving patient outcomes,” says Rivière.
- Read more articles by InterSystems on TechCentral
- This promoted content was paid for by the party concerned