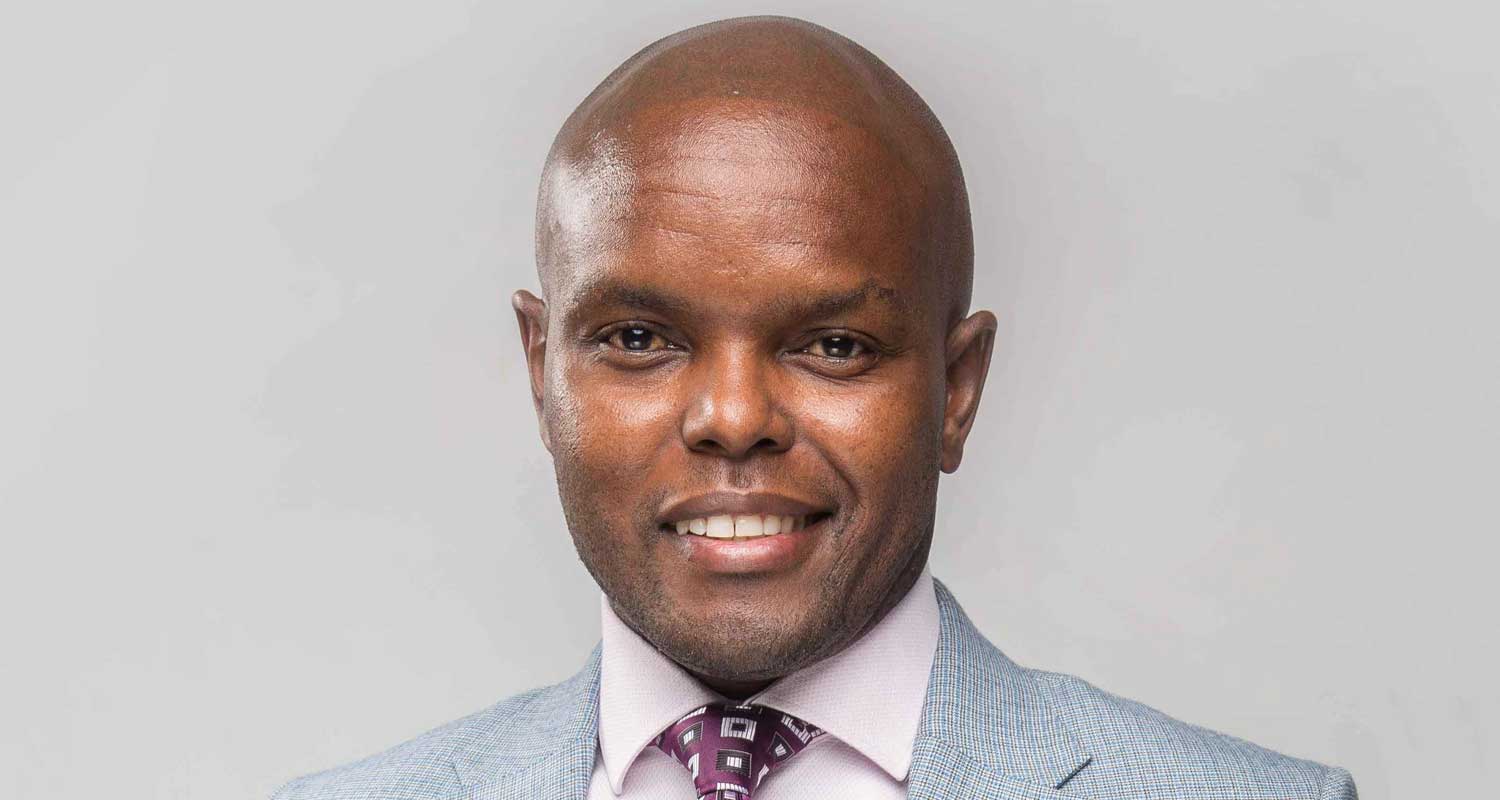
“Building AI responsibly is the only race that really matters,” Sundar Pichai, CEO of Google and Alphabet, said recently.
Responsible artificial intelligence involves designing, developing and deploying AI in a way that has good intentions, empowers employees and businesses and positively impacts customers and society. By prioritising responsible AI, companies create trust in AI both internally and externally, making it easier to scale AI systems with confidence.
Getting customers to trust in AI relies on stakeholders’ understanding that an entire organisation uses AI responsibly, rather than simply individual AI systems being deemed trustworthy or untrustworthy. Furthermore, it is the organisation’s reputation that AI systems inherit.
Deploying AI requires careful management to prevent unintentional damage to brand reputation, as well as harm to workers, individuals and society. Ethical and legal considerations are also crucial for each use case, such as obtaining consent, protecting data privacy, eliminating bias and discrimination, and ensuring the ethical use of AI for the good of the business, employees, and customers. Identifying these cross-cutting themes is essential to successfully deploying AI.
Responsible AI and governance
Responsible AI guidelines ensure that AI systems are secure, respect privacy and avoid biases. McKinsey suggests that organisations should not avoid using AI altogether, but instead focus on ensuring responsible building and application. This is achieved by ensuring that AI outputs are fair, preventing discrimination, protecting consumer privacy, and balancing system performance with transparency into how AI systems make predictions or decisions.
Although data-science leaders and teams are the experts in understanding how AI works, it’s important for all stakeholders to be involved in addressing these concerns. All employees should be aware of the ethical and legal considerations around AI and work together to ensure they are using it responsibly within an organisation.
AI developers, meanwhile, must apply responsible governance during the building phase of products and services — not just during the checking phase — to drive accountability. Data and AI governance are crucial to ensure pre-emptive safety standards in AI and data science.
To achieve this, businesses must adopt customer-focused safety standards similar to those in industries like construction and vehicle manufacturing. These standards must be integrated into the overall governance frameworks of organisations that deal with customer information. The implementation of such standards requires clear roles, responsibilities and accountability of everyone involved in the AI development and adoption value chain.
Moreover, the analytics safety standards must recognise that customers own their information and have the right to feel secure about how organisations analyse and use it. By implementing these standards, organisations can ensure the responsible use of AI and data and build trust with their customers.
The Ammanath framework
Beena Ammanath, executive director of the Global Deloitte AI Institute and founder of Humans for AI, provides a framework to ensure the ethical use of AI and maintain the trust of employees and customers. Her framework includes six steps:
- The first step is to implement fair and impartial use checks that minimise discriminatory bias and prevent unintended consequences.
- To ensure transparency and accountability, organisations must make algorithms and correlations open to inspection so that participants can understand how their data is being used and how decisions are made. However, the complexity of machine learning and the popularity of deep-learning neural networks can make this challenging.
- Policies should be established to determine who is accountable when AI systems produce incorrect results;
- AI systems must be protected against cybersecurity risks, because vulnerability is the biggest concern among early adopters of AI.
- Continuously monitoring AI systems to ensure they are producing reliable and consistent results. While the ability of AI to learn from humans is a key feature, it also introduces potential risks such as biases.
- The final step is ensuring consumer privacy is preserved and respected, that consumers can opt out at any time, and that their data is only used for purposes they’ve consented to.
Managing bias
The NIST special publication, “Towards a standard for identifying and managing bias in artificial intelligence”, points out that, while many organisations seek to utilise this information in a responsible manner, biases remain endemic across technology processes and can lead to harmful impacts, regardless of intent. These harmful outcomes, even if inadvertent, create significant challenges for cultivating public trust in AI.
“Trustworthy and responsible AI is not just about whether a given AI system is biased, fair or ethical, but whether it does what is claimed. Many practices exist for responsibly producing AI,” Schwartz et al explain. “The importance of transparency, datasets, and test, evaluation, validation and verification (TEVV) cannot be overstated. Human factors such as participatory design techniques and multi-stakeholder approaches, and a human-in-the-loop are also important for mitigating risks related to AI bias.”
There are numerous categories of AI bias, and interconnections between them. For example, there’s systemic bias (which includes historical, societal or institutional factors), which is linked to both of the other dominant categories: human bias and statistical/computational bias.
Human bias includes individual examples (like mode confusion, loss of situational awareness, or the Dunning-Kruger effect where people overestimate a technology’s abilities) and group ones (like groupthink or sunk cost fallacies).
Statistical/computational bias includes issues around selection and sampling, processing, and validation, and use an interpretation. These can include issues with data generation, representation, data dredging, feedback loops and error propagation.
To guard against these, Hall suggests seven questions to ask:
- Are the outcomes roughly equal across demographic groups no matter what the input data says?
- Do you have equal accuracy across these groups, and are you documenting what you’re doing about this?
- Is your data privacy model compliant with relevant data privacy laws?
- Have you applied what would be deemed reasonable security standards like the NIST cyber framework?
- Can you explain how your system makes a decision?
- How does your organisational chart prevent people from making bad decisions with AI?
- Are all those third parties that you’re interacting with doing all these things?
An evolving landscape
Earlier this month, Geoffrey Hinton, whose research into neural networks was pivotal to the creation of AI as we know it, and which saw him often called the “Godfather of AI”, left his role at the online search and advertising giant Google. Hinton said he left Google so that he could speak frankly about his concerns about the attendant risks of AI, rather than because of how Google is using it, but the move is concerning, nonetheless.
As technology giants like Google, Microsoft, Amazon and others seek to harness AI, a growing number of researchers and experts like Hinton are urging caution. One of the most pressing concerns is how AI can be used to create deep fakes and other misleading information, the effect it could have on employment, and the risks if applied to warfare.
Hinton highlights five ethical concerns about AI, in particular, that he believes we need to pay heed to, especially in light of the speed with which AI is evolving:
- AI surpassing human intelligence, and that generative AI like GPT-4 is already showing signs of being far more intelligent than expected.
- The risks of AI chatbots being exploited by malicious actors. For instance, using AI to create misinformation-spreading chatbots, using social media to manipulate electorates, or creating deepfakes.
- AI is increasingly able to learn from very small sample sizes, which means it’s on course to acquire skills even more rapidly than humans can, meaning it could conceivably one day outmanoeuvre us.
- The existential risk posed by AI systems, where they create their own goals and seek more power, while also being able to surpass human knowledge accumulation and sharing capabilities.
- AI and automation displacing jobs in certain industries, with manufacturing, agriculture and healthcare being particularly affected.
Pre-emptive measures
An analysis from PWC suggests organisations adopt nine, core, ethical principles to ensure their deployments of AI are responsible. These principles can be divided into epistemic and general categories which can be used to assess how ethical an AI system is and to ensure those in development result in responsible outcomes.
The epistemic principles include interpretability and robustness. That is, an AI system should be able to explain how it makes decisions. It should also be reliable, secure, and produce consistent results over time.
Meanwhile, the generic principles concern how AI should behave when contending with moral decisions in a specific cultural or geographic environment. They include accountability, data privacy, lawfulness and compliances, beneficial AI, respect for human agency, safety, and fairness.
It’s essential that businesses link ethical AI to human rights and organisational values. Connecting ethical principles to human rights can avoid regulatory ambiguity in AI development. But more importantly, incorporating human rights ideas can establish moral and legal accountability and promote human-centric AI for the greater good.
This aligns with the European Commission’s trustworthy AI ethics guidelines. Additionally, aligning ethical principles with organisational values, business ethics practices, and objectives can help create actionable AI ethics frameworks with clear accountability and monitoring methods to shape AI design and governance.
As with most new technologies, there’s the potential to use AI for good or for bad. What matters is how people and businesses choose to use it, and ensuring the necessary guardrails are in place so that AI is responsibly deployed, used, monitored, and developed, and that it creates a net positive in the world, and for humanity at large.
- The author, Prof Mark Nasila, is chief data and analytics officer in First National Bank’s chief risk office
- Read more articles by Mark Nasila on TechCentral
- This promoted content was paid for by the party concerned