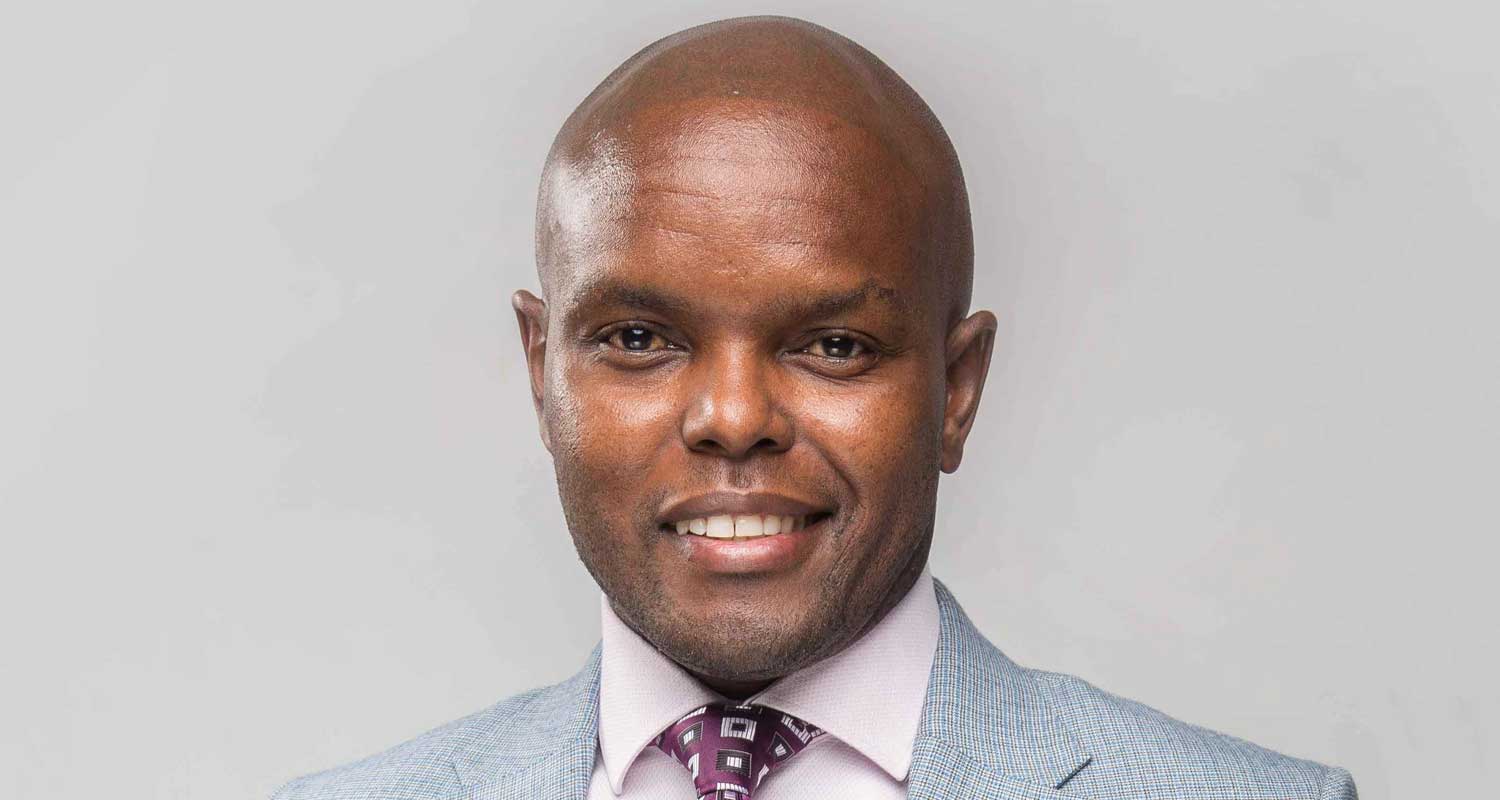
“Data is the lifeblood of any AI system. Without it, nothing happens.” — David Benigson, Signal
A data strategy outlines how an organisation will manage and leverage its data assets to achieve its business objectives. It involves defining the data architecture, governance, management and analytics practices used to ensure that data is accurate, accessible and secure.
A good data strategy should align with the overall business strategy and provide a framework for making decisions about data acquisition, storage, processing, analysis and usage. It should also address issues related to data quality, privacy and regulatory compliance. Ultimately, a data strategy aims to enable an organisation to derive insights and value from its data to support better decision-making and improve business outcomes.
Without a solid data strategy, the chance of realising business objectives with artificial intelligence (AI) and machine learning (ML) is greatly reduced while at the same time the risks are magnified. Ultimately, AI and ML depend on up-to-date, use-case-appropriate data to function at all, let alone achieve high-level business goals.
To work effectively, ML requires large quantities of quality data. To obtain this data, a process for identifying, procuring and accessing it must be established. This requires governance guidelines and a data ecosystem that supports both exploratory and production environments. But, as always, access and flexibility must be balanced with security, privacy and quality control.
“I can’t stress this enough: data or the lack of the right data strategy is the number one bottleneck to scaling or doing anything with AI,” said Nitish Mittal, a partner in the digital transformation practice at Everest Group. “When clients come to us with what they think is an AI problem, it is almost always a data problem. AI depends on viable data to prosper. That’s why it’s important to think about the data first.”
Data-centric AI
When creating a data strategy for AI, it’s essential to focus on the relevant data to fuel the appropriate use cases. It’s important to engineer the data to the use case, not merely to collate and centralise it.
Andrew Ng is the founder and CEO of Landing AI, a company trying to make no-code AI solutions, and a pioneer in the field of deep learning. In an interview with Fortune in June 2022, Ng explains how he’s become a vocal advocate for what he calls “data-centric AI.”
Ng says the availability of state-of-the-art AI algorithms is increasing thanks to open-source repositories and the publishing of cutting-edge AI research. This means businesses can access the same software code as larger companies like Nasa or Google. However, the key to success with AI is not the algorithms themselves, but rather the data used to train them. This involves gathering and processing data in a governed manner.
Data-centric AI is what Ng calls “smartsizing” data: using the least amount of data to build successful AI systems. He believes this shift is essential if businesses are going to take advantage of AI, especially those that may not be able to afford data scientists of their own or whole teams to focus on their data strategies.
Ng says companies may need less data than they think if it is prepared the right way. With the right data, even a few dozen or a few hundred examples can be sufficient for an AI system to work not just effectively, but comparably to those built by consumer internet giants that have billions of examples at their fingertips.
Preparing the data, according to Ng, means ensuring it’s “Y consistent”. That is, there should be a clear boundary for classification labels. For instance, in the case of an AI system designed to find defects in pills, labelling any scratch shorter than a certain length as “not defective” and any scratch longer than that as “defective” can help the system perform better with less training data, compared to inconsistent labelling that may introduce ambiguity or false positives or negatives.
An effective data strategy should comprise the following components: acquisition and processing, quality, context, storage, provisioning, and management and security. The strategy should involve obtaining and processing the necessary data for developing prototypes and algorithms. The data set should be of good quality with minimal bias and high accuracy labelling of training data to address business challenges.
Understanding the source and flow of data is also essential to share it effectively within the organisation. The storage of data should also be appropriate, and its structure should support the objectives concerning access, speed, resilience and compliance. Optimising the accessibility of data to the teams that need it and implementing safeguards are important too. Finally, data management and security should be in place to ensure appropriate use of datasets, including data security, access, and permissioning.
Understand data context by capturing the human elements
To make informed decisions about data usage, it is important to document the human knowledge regarding how the data was collected. This will help you make sound decisions based on the downstream analysis of the data, and helps drive explainability and accountability. A data point might be useful, but not if you don’t know where it stems from.
To ensure effective use of data, it is important to understand its provenance, including where it came from, how it was collected, and any limitations in the collection process. Consider whether the data relates to a specific group or a diverse population, and determine if any digital editing has been applied to images or audio. Each of these changes can affect its useability.
Accuracy and precision of your data matter, so it’s important to define your variables and understand the systems and mappings through which your data points have passed. Defined variables help to differentiate between raw data, merged data, labels and inferences. When processing data through multiple systems and mappings, problems can arise, causing the quality of the data to degrade over time. To avoid this, ensure that your mappings retain detail to preserve the accuracy and precision of the data throughout the process.
Generating artificial data can help fill gaps in real-world datasets and eliminate the need for potentially sensitive private data
To simplify the process of labelling data, it can be helpful to use established AI and data methods. For visual classification, a tool like ImageNet — which can identify relevant image categories and object location — can be used. By highlighting a specific area in the image, labellers can then provide more detailed classifications, such as identifying the model of a car.
To make the data labelling process easier for natural language processing (NLP), you can use existing textual content and classifiers like sentiment analysers to categorise data into general groups that can be confirmed by a person and then used for further applications.
Clustering techniques can be used to group similar data together, making it easier to label in larger volumes. Additionally, generating artificial data can help fill gaps in real-world datasets and eliminate the need for potentially sensitive private data. Gartner predicts that by 2024, synthetic data will make up 60% of all data used for AI and analytics, making it a growing area of interest.
Handling imbalanced data sets
An AI-powered solution is only as good as the source data it’s fed. Faulty data leads to faulty outputs. One of the leading sources of inadequate results are imbalanced data sets. For instance, if a particular group is over-represented in a dataset, it can lead to minorities being overlooked or their needs being inaccurately predicted. There are various sorts of imbalances — including intrinsic and extrinsic ones — and various methods, eg, over-sampling, under-sampling, synthetic minority oversampling technique, and generative adversarial networks that can be explored to overcome them.
To successfully create an AI strategy, it’s imperative to have an equally robust data strategy that removes complexities, aligns data with business objectives, is constantly checked and adjusted to mitigate bias or other failings, and which those responsible for data collection and management in the business buy in to and support. Without data, there is no AI, but with it, the possibilities are nearly limitless.
- The author, Mark Nasila, is chief data and analytics officer in FNB’s chief risk office
- Read more articles by Mark Nasila on TechCentral
- This promoted content was paid for by the party concerned