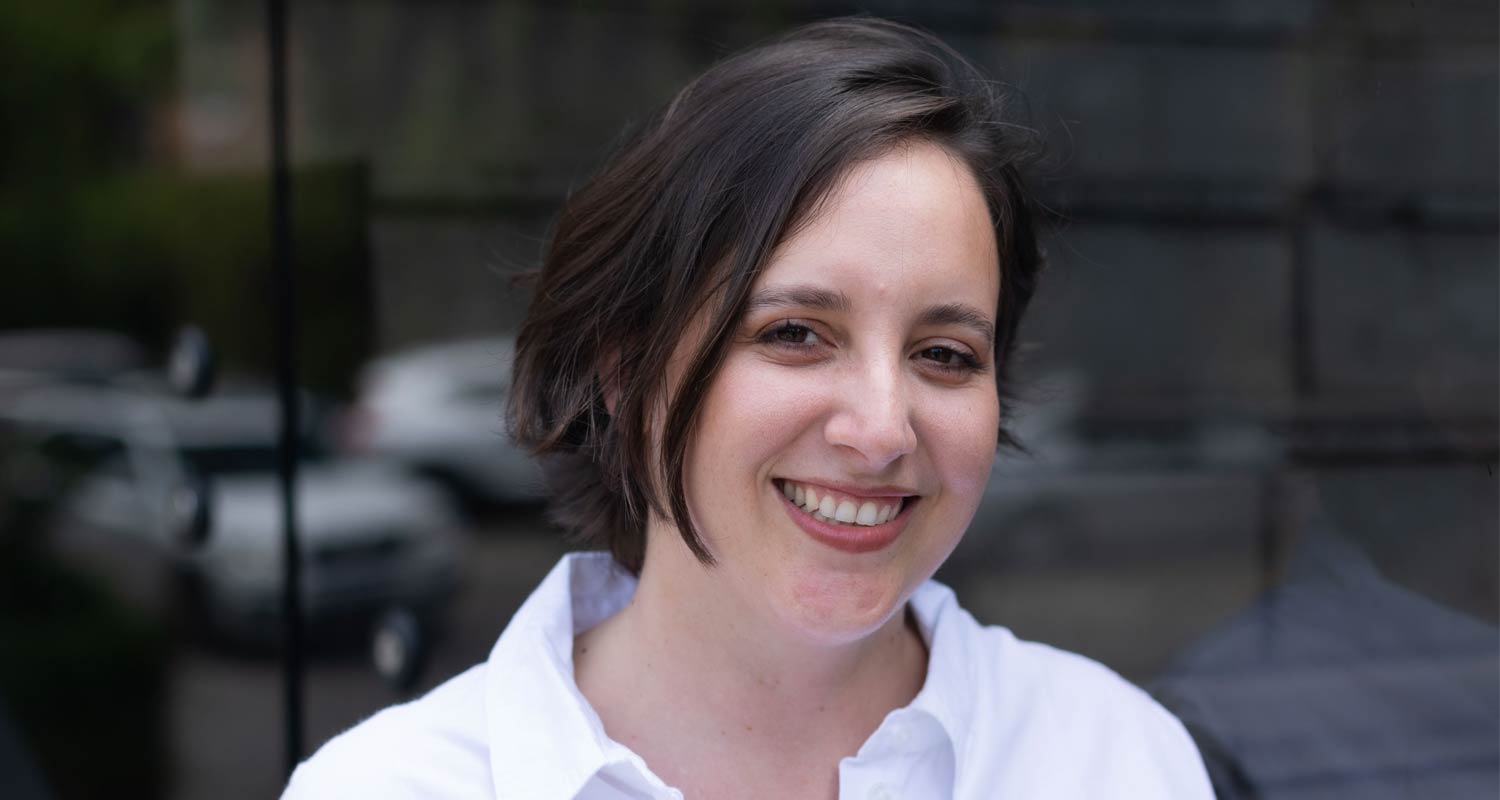
New language tools are being built that allow speakers of indigenous African languages to interact with the latest artificial intelligence applications.
Lelapa AI is a local AI research and product lab that is building “language technology”, including large language models (LLMs), using indigenous African languages such as isiZulu and seSotho, to help speakers of these languages interact with the latest tools.
TechCentral spoke with Jade Abbott, chief technology officer at Lelapa (the seSotho word for “home”), to learn more about the challenges and opportunities in the natural language processing (NLP) space.
“The internet is over 90% English; this means that only certain parts of the world have access to this powerful tool,” said Abbott. “We need to build the language technology that ensures we are represented as a continent, that makes digital knowledge and services accessible to us.”
But building NLP tools for indigenous languages is not as easy as it is for languages such as English and French, Abbott said. Described by NLP experts as “high-resource” languages, French and English have large data sets available on the internet that can be “scraped” and used to train new NLP tools. In contrast, “low-resource” languages such as isiZulu and seSotho do not have vast data sets available for scraping, which makes developing computational tools for processing these languages more difficult.
‘Do it from scratch’
To get around this problem, Lelapa uses a “do it from scratch” approach and creates the data required to train the models that they produce. This methodology has its own complexities:
- Firstly, languages are large and nuanced, so training models on them requires massive data sets;
- Secondly, the computing capacity required to train these models is vast and therefore costly; and
- Thirdly, the standard tools used to evaluate the efficacy of language processing tools work well for languages like English but are less useful for indigenous languages.
Lelapa employs various strategies to get around these complexities. The first involves shrinking the application domain for the model being built so that the resulting model is as small at it can be to solve the problem being addressed.
This has the added benefit that the compute resources required to build the model are also minimised, which drives down costs.
“We build our models similarly to how an engineer might build a bridge,” said Abbott. “We know exactly how well the model works within a specified domain and what the tolerances are. We don’t try to build a generalisable tool that is going to work everywhere because there is not enough data – it is not going to work.”
The specified domain can be finance or agriculture, for example. But Lelapa also makes use of native language speakers throughout the development process to ensure its models are accurate. This is especially important in the evaluation phase of the process, where standardised tools such as the Bleu score are not as effective for indigenous languages.
A third component of Lelapa’s development strategy is to use tools that fit the problem, a methodology that sometimes leads to the exclusion of AI in lieu of a more straightforward computational solution, said Abbott.
“When the application domain is well understood, you sometimes don’t want to add a generative tool because of the complexity that comes with that,” she said.
According to Abbott, the company is seeing most demand for its transcription and conversational products. Lelapa tools are being used in the financial sector where clients such as banks are able to coax their less digitally savvy clients onto digital platforms knowing that the customer support for these apps can be facilitated in the customer’s native language wherever it is needed.
Call centres are also making use of Lelapa’s tools, especially for quality control, where AI is being used to evaluate interactions between agents and customers to ensure that company representatives are “not overpromising” in sales calls to non-English speaking clients, for example.
Read: Google apologises for ‘woke’ AI tool
“Before deciding on using these tools, companies must evaluate how well they work for their specific use case and see how it will augment their people rather than replace them. We are still a long way off from AI being powerful enough to replace humans, but carefully considering how it might augment workers will help derive more value from it,” said Abbott. – © 2024 NewsCentral Media