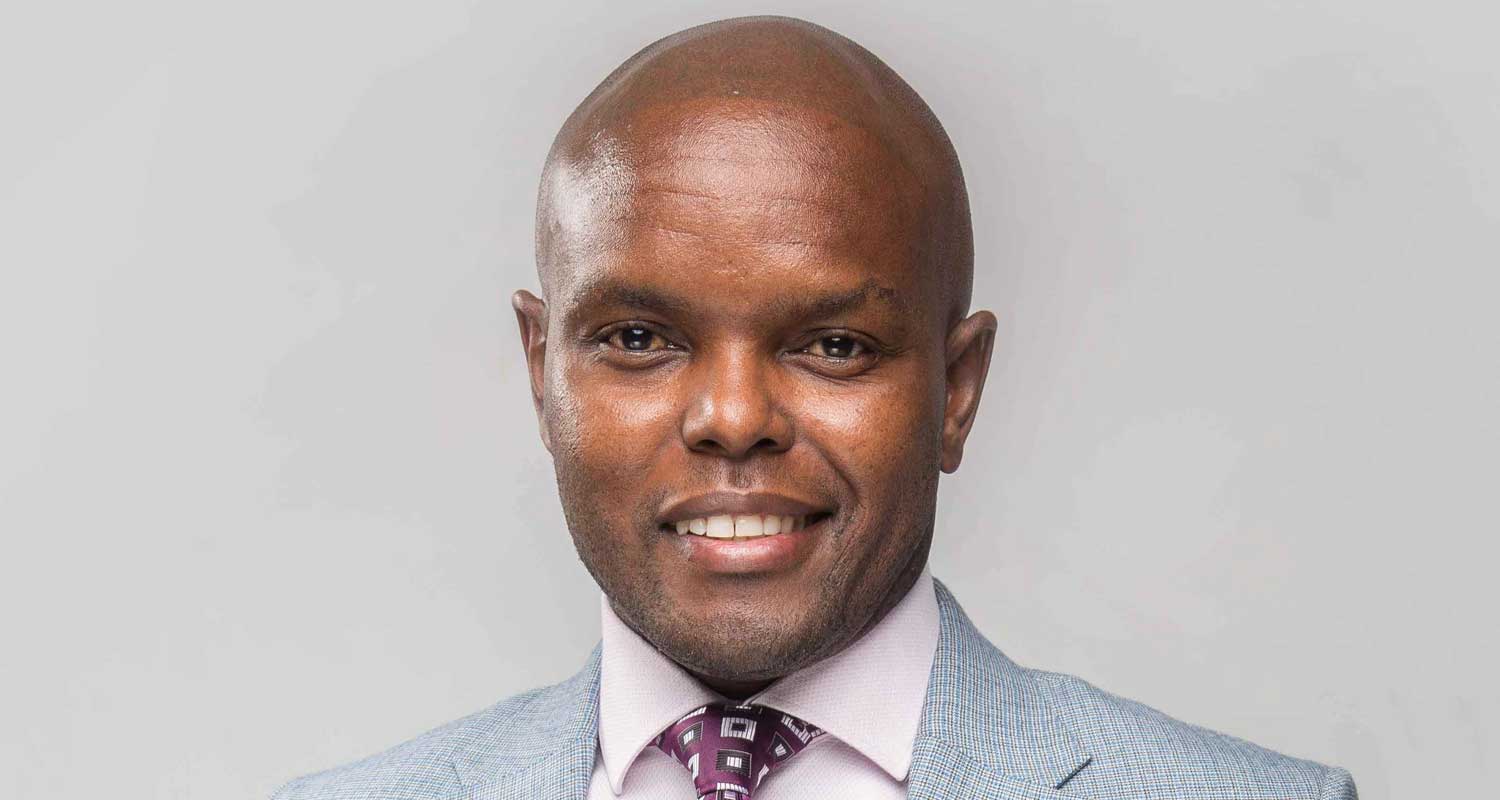
Becoming an artificial intelligence-driven organisation means fully digitising business processes. It starts with using AI to augment or automate tasks. But to get to that point, it’s essential first to establish a culture where data is trusted. That means business-wide data literacy, and appropriate data collection, sorting and storage solutions – because, if teams can see the value they can derive from data, and that the data is sound, it makes trusting that data to AI all that much easier.
Managing expectations about AI’s capabilities is important at the outset of an AI adoption journey through AI literacy. This starts with identifying appropriate use cases – activities where AI can reasonably be expected to be effective and demonstrate success, which can then be adapted to other processes and encourage support from other sectors of the organisation.
It also means starting with the outcomes in mind and understanding the organisation’s priorities, goals and customers. In this way, an organisation is better positioned to create a practical AI strategy that can demonstrate its value sooner, and likely be monetised earlier.
It often makes sense to start with a clear task that’s very data dependent and for which the necessary data exists in the organisation already. By bringing on experts to help find that use case, it can be implemented as a proof of concept to reduce resistance. Thereafter, the organisation can identify subsequent projects that can benefit from similar AI implementation, whether trimming costs in a particular activity, reaching consumers in new ways or reducing repetitive work.
“Understanding the potential of AI (both good and bad) starts by understanding how AI works and what it can really do,” says Bill Schmarzo, dean of big data at Dell Technologies. “That’s probably our best chance to ensure that AI works for us versus us working for AI.”
Some potential use cases include developing more intelligent products or services, automating repetitive tasks, or manufacturing processes, improving data security and risk estimation, predictive analysis to identify trends, or creating personalised customer experiences via marketing efforts or support services.
Detailing an organisation’s potential use cases makes it possible to rank them by importance. This is because at first it’s unlikely a business will be able to do all of them, so instead it can pick some of the manageable ones with which to start. It may also be sensible to pick those most likely to get buy-in from leadership, and those with short-term horizons so that value can be demonstrated as swiftly as possible.
Choose the right metrics
It’s equally important to choose the right metrics to use to make informed decisions, and which to use to assess success. One way to help create metrics is to understand how the organisation creates value, and to identify the KPIs against which to measure effectiveness. AI is a tool, which means there’s the potential for misuse, or for trying to use it to solve a problem for which it isn’t suited, only to be disappointed when it fails.
Work by Schmarzo has found that it’s extremely difficult to understand the value AI can bring if we don’t understand how an organisation creates value in the first place. But without that understanding, it’s impossible to create AI solutions that will bolster that value.
The right strategy
An easier way to understand metrics is to understand the type of AI strategy an organisation is trying to achieve through a use case. A use case might be trying to drive efficiency, effectiveness, expert strategy or innovation. Metrics should be derived through one of the four or result from one of them. For example, “the efficiency strategy” metrics will differ from those for other strategies.
Examples of the efficiency strategy include factory work, where robots take over activities on a factory assembly line entirely. An effectiveness strategy includes things like AI systems that can generate risk assessments of financial services based on the historical data the business has about them, leaving analysts to interpret those reports but freeing them up from their time-consuming creation. Both the efficiency and effectiveness strategies are instances of automation: AI taking over roles, wholly or partially.
On the other end of the spectrum are the expert and innovation strategies, which offer augmentation rather than automation. For example, AI diagnostic tools used by doctors can compare a patient’s data to big data sets in mere seconds and offer suggestions for further testing based on its finding. Here AI augments the doctor’s expertise. Similarly, the innovation strategy could be used by a composer where AI fills in missing parts of a score or offers suggestions for melodies, furthering the creative possibilities for the composer.
Which strategy to adopt
Activities with low data complexity tend to be clearly structured and simple and depend on simple strings of characters or numbers. This sort of data is easy for a computer to parse, and adheres to rules with few deviations, meaning it also has low work complexity.
High data complexity, meanwhile, tends to be unstructured and more open to interpretation. Multimedia content like images, video, and audio are great examples of complex data. Though AI tools to identify or categorise these sorts of data are improving by the day, human intervention is often still required.
Where low work complexity means clearly defined rules and routines, high work complexity is the opposite and refers to activities with lots of deviations, variation and low levels of predictability.
That’s not to say this is a one-size-fits-all solution. As AI technology improves the range of complexity, it’s able to contend with improvements; activities too difficult for it to automate today may not remain so in future. But considering which strategy to start and which outcomes are desired is a useful starting point.
AI is a continuous endeavour
Use cases are not a once-off thing. Once applying AI to a use case has demonstrated results, it’s an opportunity to re-use that framework for similar use cases. That means progress can be cumulative, rather than having to build AI solutions from scratch for each new deployment. At the same time, the original use case may evolve, and require the AI solution applied to it to evolve, too.
But once a concept has worked, that’s an opportunity to customise and improve it. Iteration can bring unique advantages. Often the first version of the model will be very generic — it may even be off the shelf — but by making it unique, a business not only makes it better suited to its needs it also makes it more difficult for competitors to replicate.
- Prof Mark Nasila is chief data and analytics officer in FNB’s chief risk office. Read more articles by him on TechCentral
- This promoted content was paid for by the party concerned