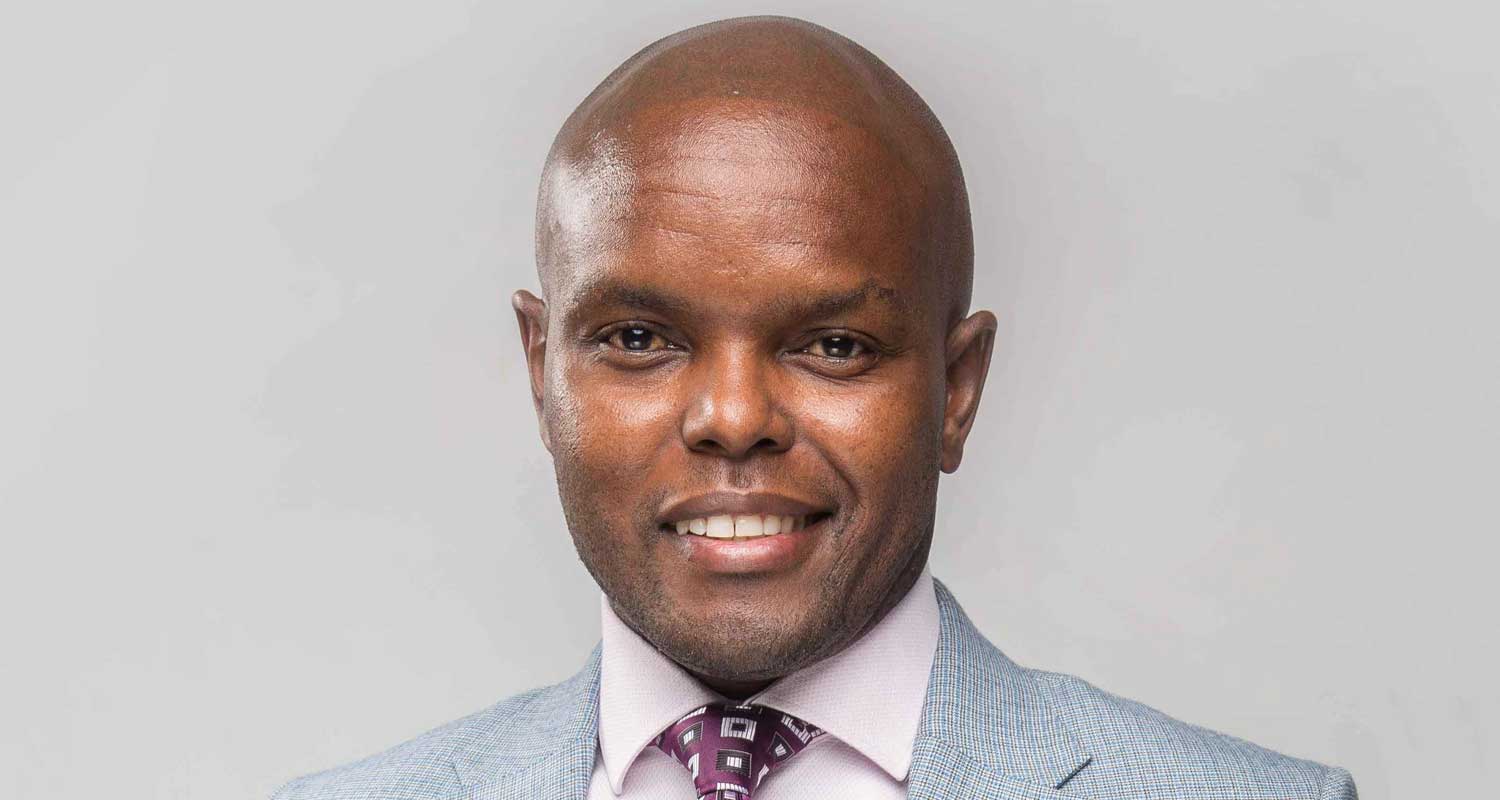
Artificial intelligence is revolutionising various industries around the world.
In China, AI is being used to educate students in over 1 700 schools across 200 cities. In Australia, an AI has developed a flu vaccine that surpasses the effectiveness of all existing vaccines. In the US, a machine-learning robot is independently preparing burgers.
Resourceful developers are already using OpenAI’s groundbreaking language-generating algorithm, GPT-3, to automatically produce popular blog posts, design websites and create interactive role-playing experiences. These are just a few examples of the incredible real-world applications of AI.
But the effectiveness of an emerging technology depends on its utilisation. As businesses aim to take advantage of innovative solutions driven by AI, they must enhance their approach to implementing technology, and they need to avoid one of the most common missteps: focusing on strategy and not implementation.
According to a Forbes report on AI business adoption in 2020, the percentage of companies investing over $50 million in Big Data and AI initiatives has increased to 64.8% in 2020 from 39.7% in 2018. Nearly all companies (98.8%) are investing in these areas. However, only 51.9% of companies are accelerating their investment rate, a significant drop from 91.6% in 2019. Additionally, only 14.6% of companies have successfully deployed AI capabilities at a widespread production level.
The primary hurdle in the adoption of AI in business lies in the complexity of organisations, which poses challenges when it comes to integrating and implementing AI solutions. High-performing companies, as highlighted in a McKinsey survey, credit a significant portion of their profits to the effective integration and utilisation of AI. This is why successful implementation of AI requires careful planning and a consistent strategy.
Focus is key
McKinsey consultants Tim Fontaine, Brian McCarthy and Tamim Saleh recommend an approach where businesses focus on using AI to enhance a specific crucial business process or function, rather than attempting to implement AI across the entire company.
It is important for businesses to rethink their business models to fully leverage the benefits of AI, as simply plugging AI into existing processes may not yield the desired results or valuable insights.
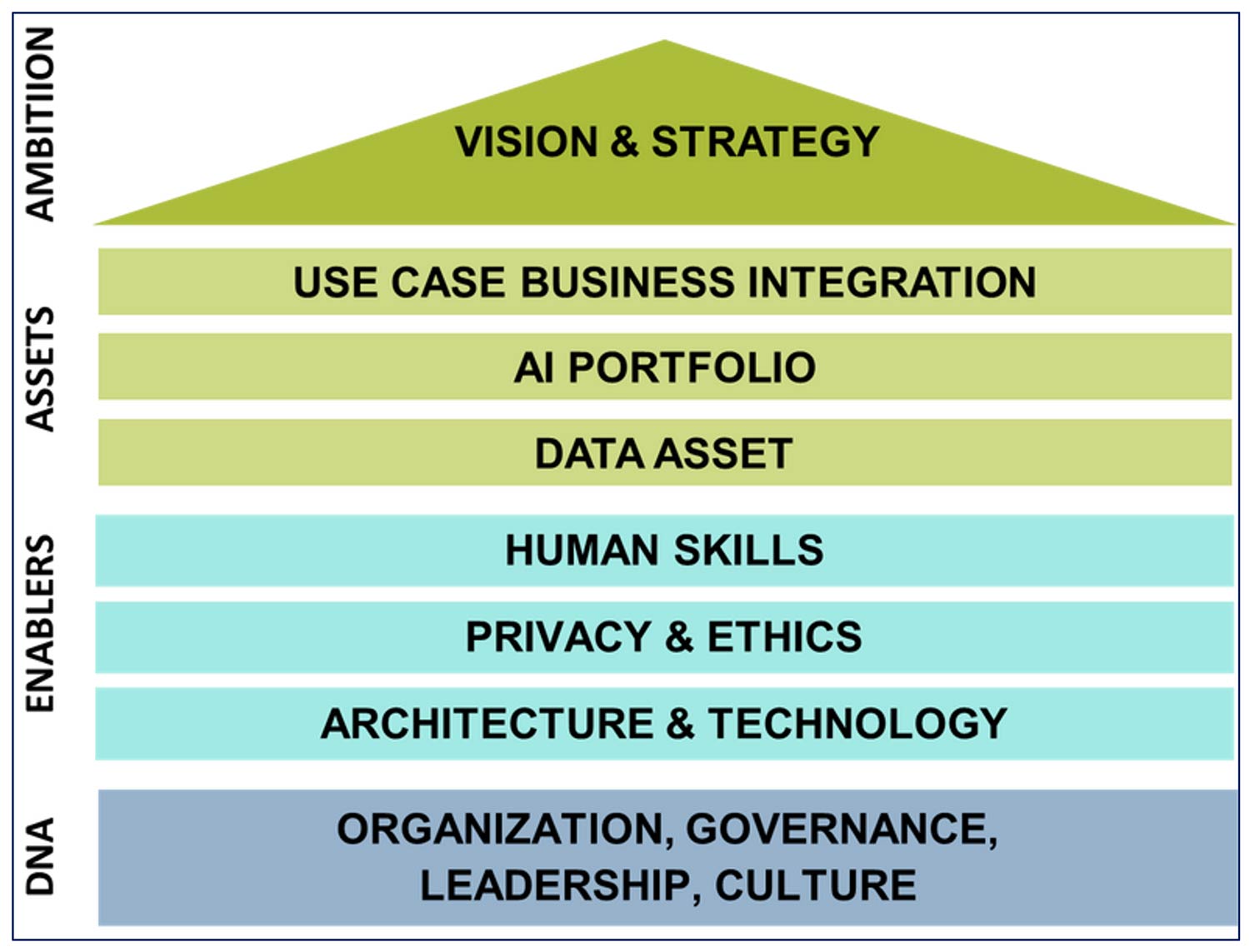
If you define the wrong use cases for AI, your organisation’s ambitions for it will be set too low, and outcomes are likely to disappoint, making further implementations harder. It’s important to start by setting an appropriate vision and strategy, and by assessing your existing internal assets, tools, capabilities, enablers and other factors that can assist with successful AI roll-outs.
Assets are a combination of data, your AI portfolio, and your particular business’s use case integration. Enablers are your human skills, privacy and ethics, your architecture and technology. Lastly, your DNA is your organisation, governance, leadership and culture.
Formulate your data strategy
At the outset, it’s essential to align your business and digital strategy with a data and AI vision, focusing on key opportunity areas for optimisation and innovation. It’s equally important to identify business processes where data and AI can be applied, including product development, sales, HR, and more – that is, to understand the current state of your organisation by assessing readiness of your current data and AI capabilities.
It pays to make sure both your assets and enablers as per Kruhse-Lehtonen and Hofmann’s framework above are set up for success, as this helps to define the target state for your processes after implementing data and AI.
Articulating the role of AI
To achieve success in implementing AI, it is crucial to develop a well-defined business strategy that aligns with specific objectives and measurable key performance indicators. A notable example is Amazon, where Jeff Bezos assigned managers in 2010 to explore how AI and machine learning could enhance their competitiveness. This strategic move played a significant role in Amazon becoming a global leader in AI implementation.
Although your journey towards AI success may differ, it is advisable to pause and evaluate your business requirements in order to chart a path towards AI transformation that’s based on solid and applicable use cases.
The selection of an appropriate AI strategy for a specific activity depends on two factors: data complexity and work complexity.
Based on the analysis of complexity, the following AI strategies are suggested for different scenarios: The efficiency strategy is suitable for activities with low data and work complexity. The effectiveness strategy is appropriate for activities with high data complexity but low work complexity. The expert strategy is recommended for activities with low data complexity but high work complexity. Finally, the innovation strategy is the preferred approach for activities with both high data and work complexity. By understanding the complexity of an activity, one can determine the most suitable AI strategy to adopt.
Hiring the right people is key
In a Forbes report on AI business adoption in 2020, 37% of respondents identified limited AI expertise or knowledge as a barrier to successful implementation. Other challenges included increasing data complexities and siloed data (31%) and a lack of tools for developing AI models (26%).
One important factor for successful AI implementation is ensuring that employees are prepared to work with smart systems. Without proper training and support, the scalability of AI solutions within businesses can be hindered. It is also essential for companies to recognise the need to update traditional policies and provide enhanced business support to accommodate AI integration.
Creating a diverse and multi-disciplinary AI team is crucial, incorporating roles such as AI and machine learning engineers, data scientists and web developers to leverage various technologies required for AI projects. Outsourcing a portion of AI development to software development outsourcing firms may also be an option if local expertise is not readily available.
Reimagine business models
It is common for businesses to adopt a product-centric approach in AI product development, where the focus is on building the product first and then finding customers for it.
A customer-centric AI strategy emphasises the development of AI solutions that improve interactions and service delivery to customers. The goal is to eliminate friction, personalise engagements and enhance overall efficiency, leading to increased revenue and profitability.
By aligning the AI strategy with customer-centric key performance indicators, businesses can measure the results and identify ways to better serve their customers across various aspects of their operations. Adopting a customer-centric approach in AI strategy allows businesses to prioritise customer needs and preferences, ultimately driving success in the market.
Select suitable technologies
It is advisable for companies to identify AI technologies that can accelerate the creation of new business capabilities and subsequently allocate further investments to other prioritised areas within the company.
The term “technical debt” is commonly used to describe the impact of choosing an easy solution in software development, which leads to additional rework and costs compared to a better approach that may take longer. According to a McKinsey survey, CIOs reported that a significant portion of the technology budget dedicated to new products is diverted to resolving issues related to tech debt. They estimated that tech debt accounts for a substantial percentage (20-40%) of the value of their entire technology stack.
If an AI program fails to be accepted by users, it becomes a once-off solution instead of a learning system
In addition to technical debt, there are also other forms of debt, such as people debt, caused by supporting outdated assets and resulting in employee attrition; and process debt, which refers to work that doesn’t add value to the organisation or its goals.
Scalability and upgrading considerations
AI and data science rely on continuous learning and feedback for development. If an AI program fails to be accepted by users, it becomes a once-off solution instead of a learning system. To promote adoption, businesses can use immediate feedback from MVPs or prototypes, provide employee training, and conduct review sessions for ongoing improvement. Any successful AI solution for a specific business problem, no matter how small, can showcase the advantages of AI and build support for larger scalable projects.
AI should be treated as an ongoing effort by businesses rather than a one-time endeavour. This means continually investing resources to improve and refine the AI program, making it difficult for competitors to replicate and strengthening the organisation’s competitive advantage over time.
Scaling up in-house AI capabilities and fostering a culture of continuous learning and improvement are also crucial for successful AI implementation.
- The author, Prof Mark Nasila, is chief data and analytics officer in FNB’s chief risk office
- Read more articles by Mark Nasila on TechCentral
- This promoted content was paid for by the party concerned