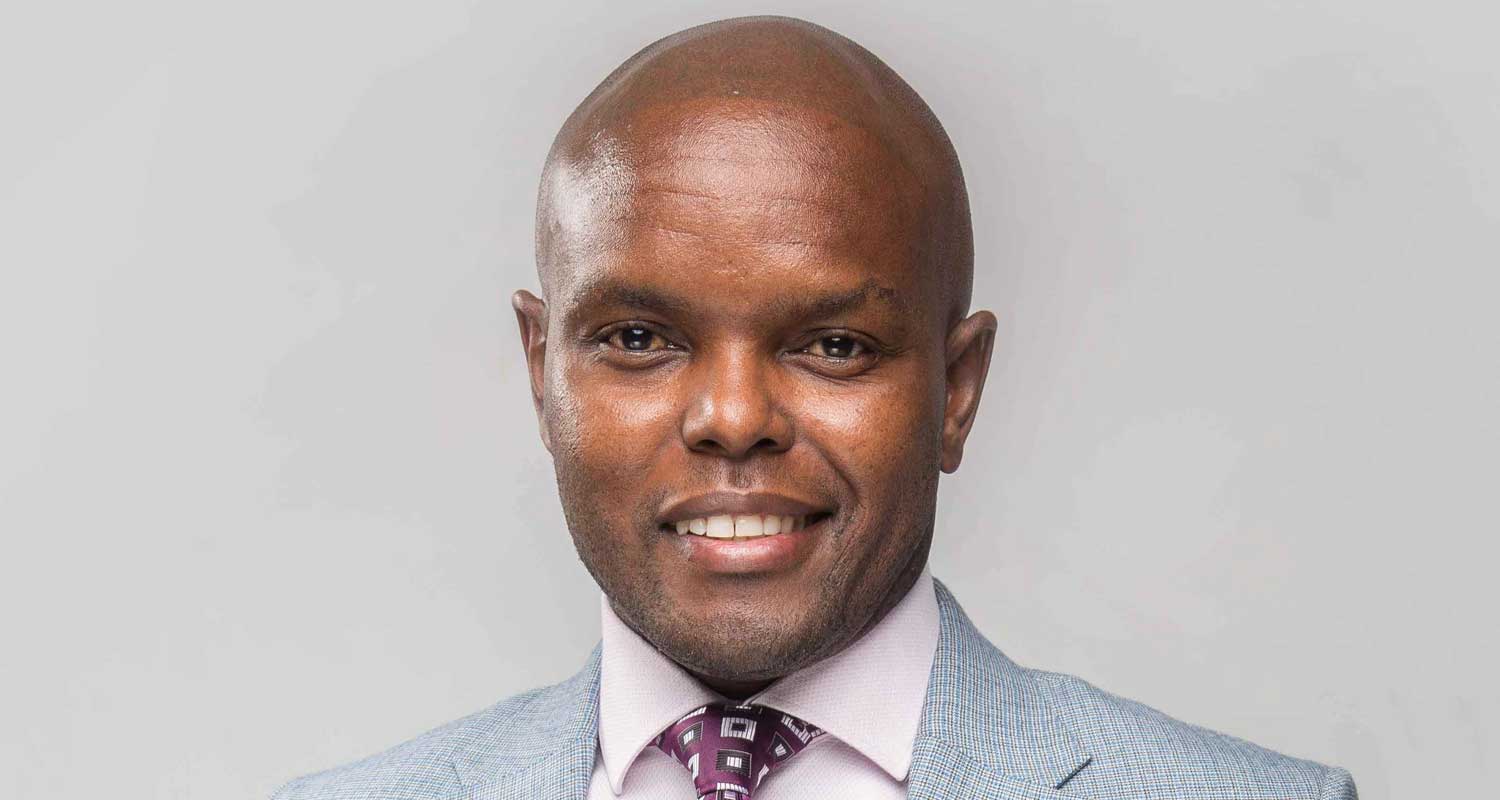
Before implementing an artificial intelligence strategy, it pays to assess your organisation’s AI readiness.
Take, for instance, commercial air travel. Having the passengers, crew and pilots on boards, along with the luggage and catering supplies, isn’t enough to fly. Pilots conduct a flight readiness review (FRR) before every flight where they assess their readiness to start and execute flight tests or operations. This includes a self-assessment tool, known as the I’m Safe checklist, to evaluate their physical and mental health status before a flight. This checklist determines the overall preparedness for a flight, as well as flight control systems and other hardware checks.
Like flight, AI offers huge potential, but there are also checks and measures required to make sure it operates as intended. Due to its inherent complexity and new organisational requirements, adopting AI can be challenging. Assessing an organisation’s readiness is crucial for successful AI adoption and leveraging its business value.
Despite many organisations including AI in their strategic plans, the majority of AI initiatives fail to meet expectations and provide real value. This is often due to a lack of foundational support for AI capabilities, resulting in isolated projects that do not integrate well with business operations.
According to a Forbes report from 2022, between 60% and 80% of AI projects fail. What sets the remaining successful implementations apart? They only go ahead when AI organisational readiness has been confirmed.
The AI Readiness Assessment is a thorough toolset that aids organisations in understanding the AI landscape and evaluating their preparedness for AI integration across diverse sectors, and can be applied to the private sector, too. The framework emphasises the organisation’s dual roles as both promoters of technological growth and users of AI in public services.
Organisations, nations and other AI users are at various stages in their AI evolution, necessitating careful evaluation to determine their specific needs and capabilities for digital infrastructure, governance and a supportive community.
Some of the existing frameworks used to measure AI readiness include:
AI Readiness Index by AI Singapore
AI Singapore has also developed the AI Readiness Index (AIRI), a tool to gauge an organisation’s preparedness to embrace and apply AI technologies. It’s proven so successful it’s since been adopted by 29 other countries.
This index assesses readiness across five pillars: organisational, ethics and governance, business value, data, and infrastructure. Under each pillar are four levels of AI readiness: AI unaware, AI aware, AI ready, and AI competent. For instance, when assessing organisational AI readiness, it may emerge that the organisation has a rudimentary knowledge of AI (AI aware) but doesn’t have the capabilities, skills or resources to implement it.
Assessing readiness in these sectors can help a business identify blind spots and address them before embarking on a potential costly AI implementation strategy that may otherwise fail.
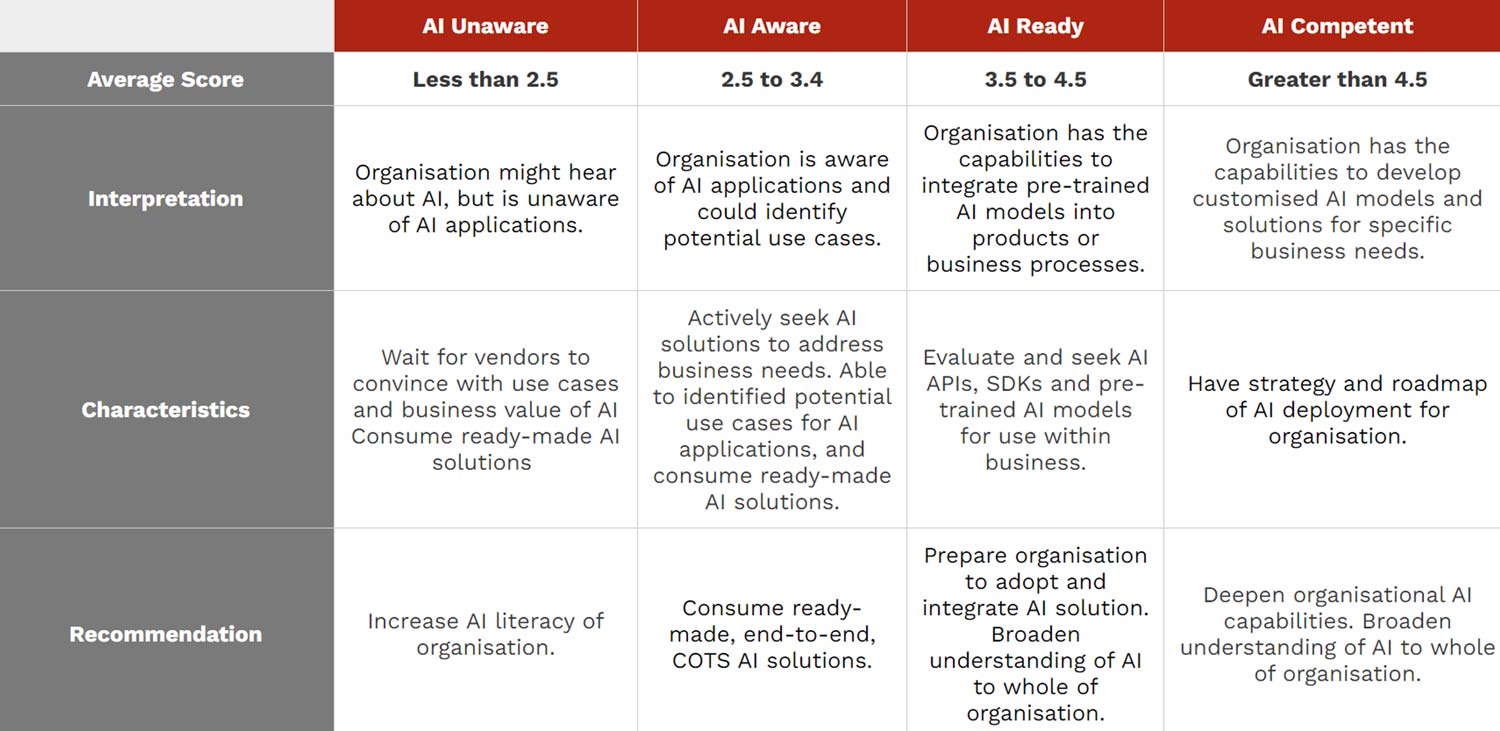
AIRI by Antonio Grasso
Grasso’s approach features four pillars and nine dimensions. The four pillars are: organisational readiness, business value readiness, data readiness and structural readiness. Let’s break each of these down.
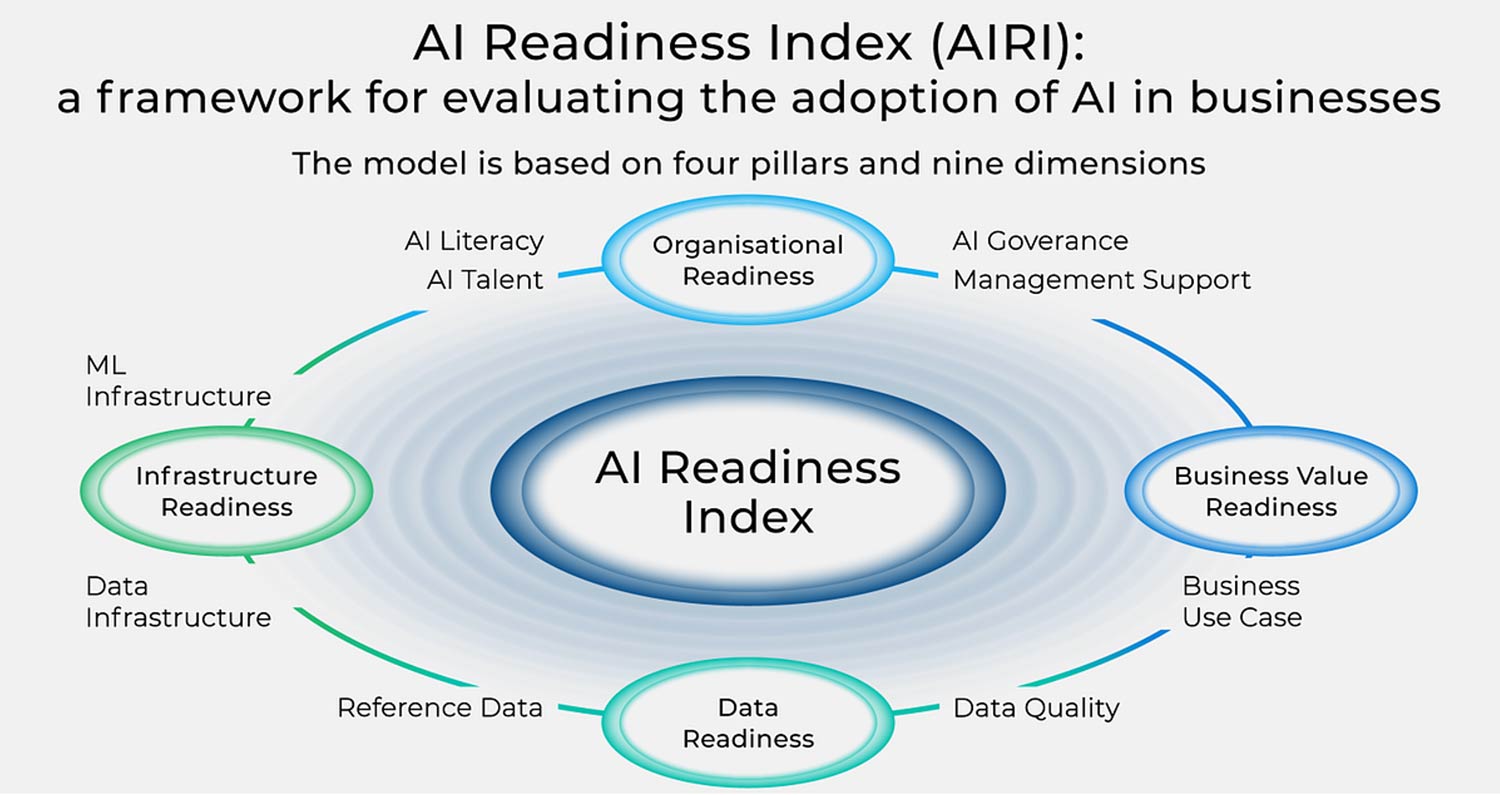
- Organisational readiness: Organisational readiness involves assessing if a company is prepared to adopt AI. This stage analyses the readiness of the company’s human resources, its skills and attitudes across four dimensions: AI literacy, AI talent, AI governance and management support, which verifies if the management is ready to back AI initiatives by providing the necessary resources for in-house development. The objective of this stage is to comprehend whether the organisation has the essential foundation to commence the journey towards AI implementation.
- Business value readiness: In this stage, the key dimension is considering business use cases: have practical, real-world applications been established? Recognising the areas that will be influenced by innovation and the specific use cases in advance can make the process of adopting AI more efficient. It can be helpful to consider the value proposition, that is, to identify where AI will bring the greatest efficiency. For instance, if the goal is to enhance customer service, an intelligent virtual assistant could be implemented. By identifying these elements beforehand, the implementation steps can be outlined more effectively, thereby improving overall process efficiency.
- Data readiness: To succeed with AI, an organisation needs the right input data. This stage assesses an organisation’s preparedness to manage the data that will be used to feed the algorithms.
- Data quality: Does the organisation have procedures to ensure the completeness and accuracy of the collected data?
- Reference data: Does a unique, standardised reference exist for the data?
- Structural readiness: This asks if your organisation has the infrastructure to support data storage, retrieval, and AI model training. It requires assessing two dimensions
- Data infrastructure: Including servers, storage, organisational processes and data management policies, often associated with data governance.
- Machine learning infrastructure: Covering human resources, processes and tools needed for machine learning models, also known as AI infrastructure or MLOps, which ensures reliable and efficient model deployment and maintenance.
- Final assessment: To create a final score, points are assigned for each of the above categories using the assessment table below.
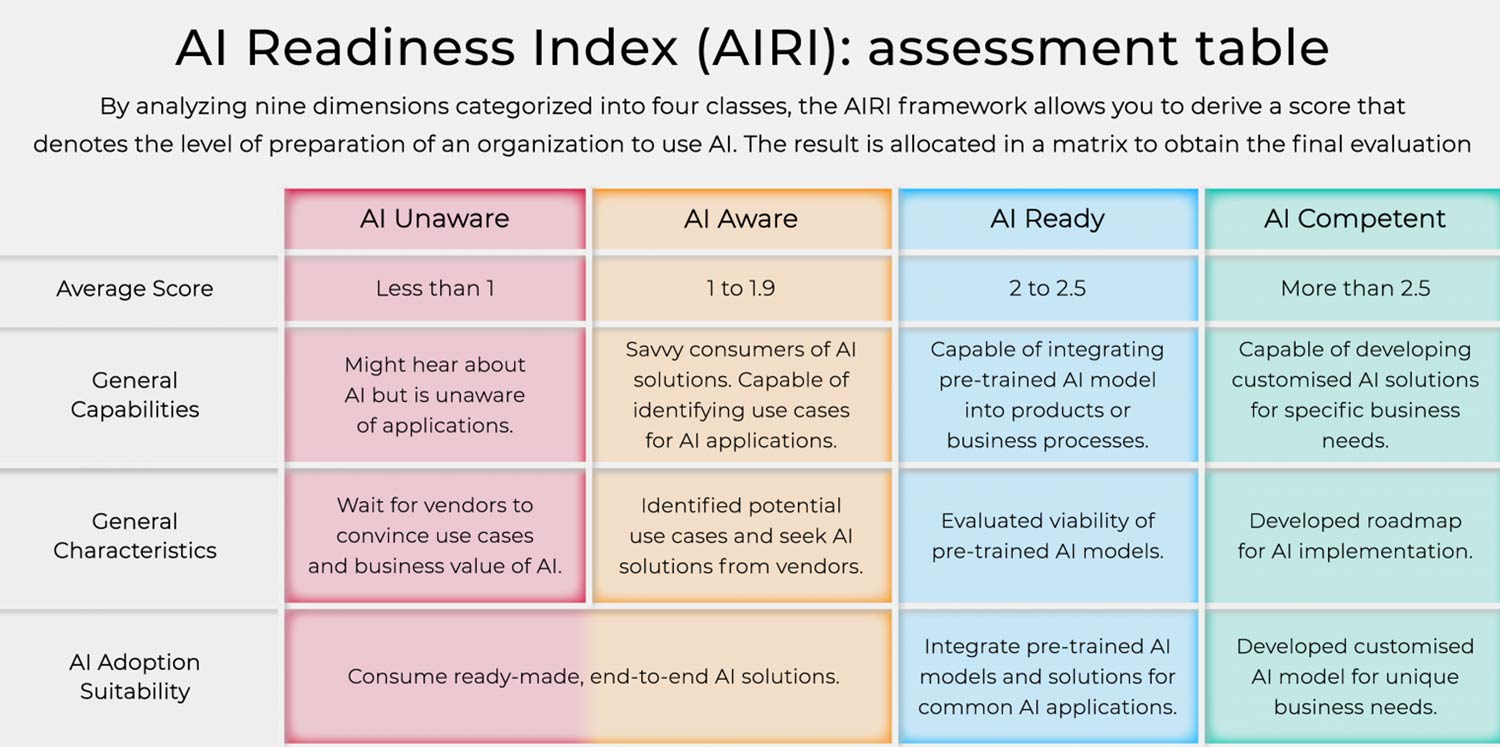
UNDP and Oxford Insights AI readiness assessment for countries
Evaluating a country’s AI readiness is a crucial initial step towards adoption that can help lessen potential risks. The UNDP AI Readiness Assessment is a comprehensive toolkit that enables governments to grasp an overview of the AI scene and evaluate their AI preparedness across different sectors. This framework mainly considers governments as facilitators of tech advancement and AI users in the public sector, with an emphasis on ethical considerations surrounding AI use.
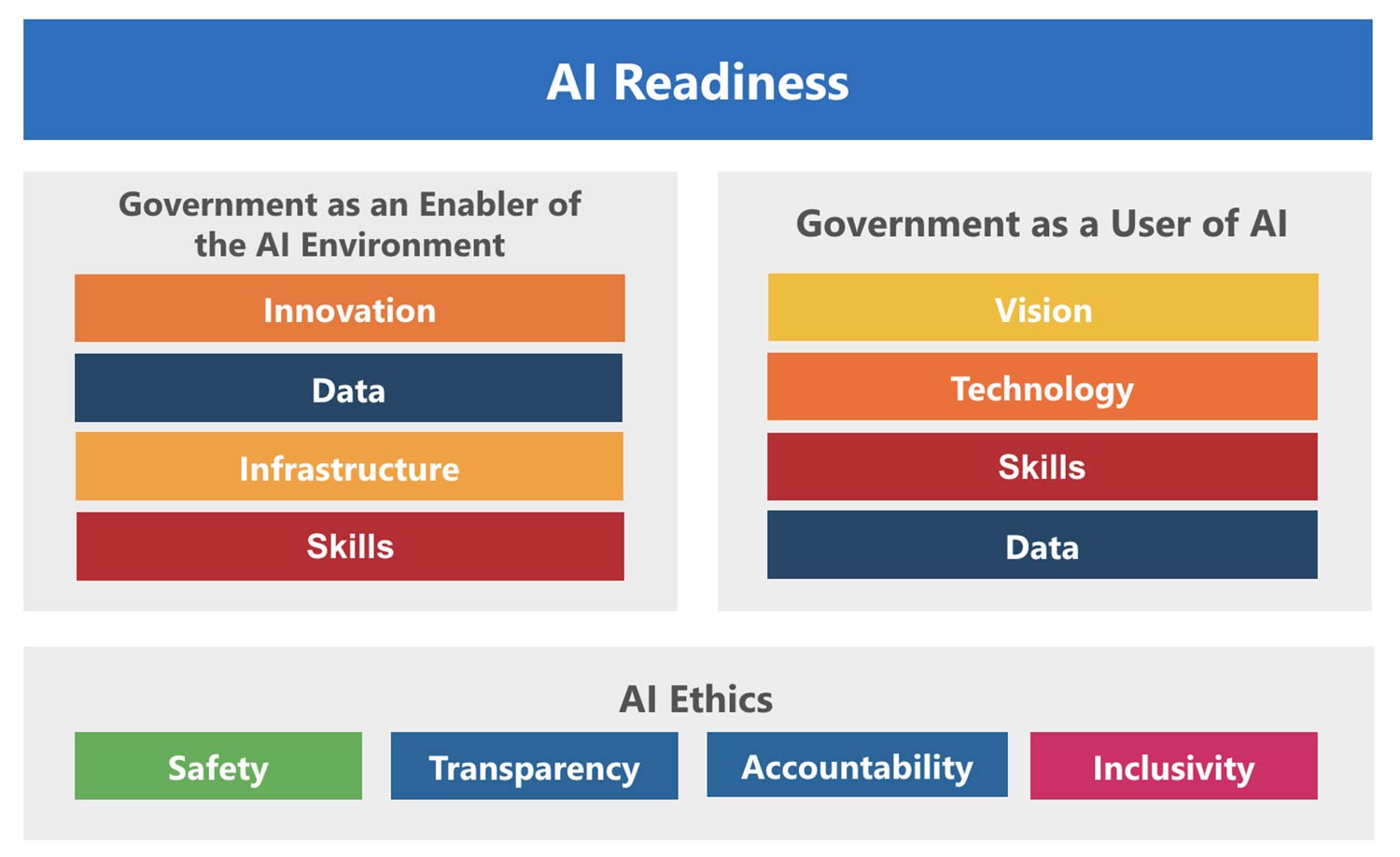
Key elements needed for ethical AI development and implementation, such as policies, infrastructure and skills, are highlighted in the assessment. These are significant for countries as they deploy AI technologies at a national level to achieve sustainable development goals.
Using a qualitative approach, the assessment utilises surveys, key informant interviews and workshops with civil servants to gain a more profound understanding of a country’s AI ecosystem. It offers governments important insights and recommendations on implementing ethical and effective AI regulations, including how AI ethics and values can be integrated into existing frameworks. Importantly, this globally applicable UN tool is available particularly for governments at any stage of their AI journey.
Deloitte’s AI readiness for government
With reference to Deloitte’s AI readiness framework – If the goal is to move beyond pilot stages, it’s beneficial to consider several interconnected areas to evaluate AI readiness. These include:
- Strategy: To develop an AI vision that aligns with organisational objectives);
- People: Organisations might struggle with sourcing necessary technical skills and helping current employees develop AI skills;
- Processes: Defining processes, controls and governance systems to facilitate successful AI implementation;
- Data: AI’s efficacy relies on the quality of data it’s built on, and it demands a lot of data;
- Technology and platforms: Procuring and developing suitable AI technology and platforms to operationalise AI assets, including considerations relating to vendors and interoperability; and
- The ethical implications of this transformative ability: To implement mechanisms to comprehend and counteract AI bias, encourage fairness and transparency, and embed values and integrity into AI initiatives).
These six areas are crucial as all of them will likely need attention and modification during an agency’s AI journey. They can help establish a starting point for your readiness to undertake this journey.
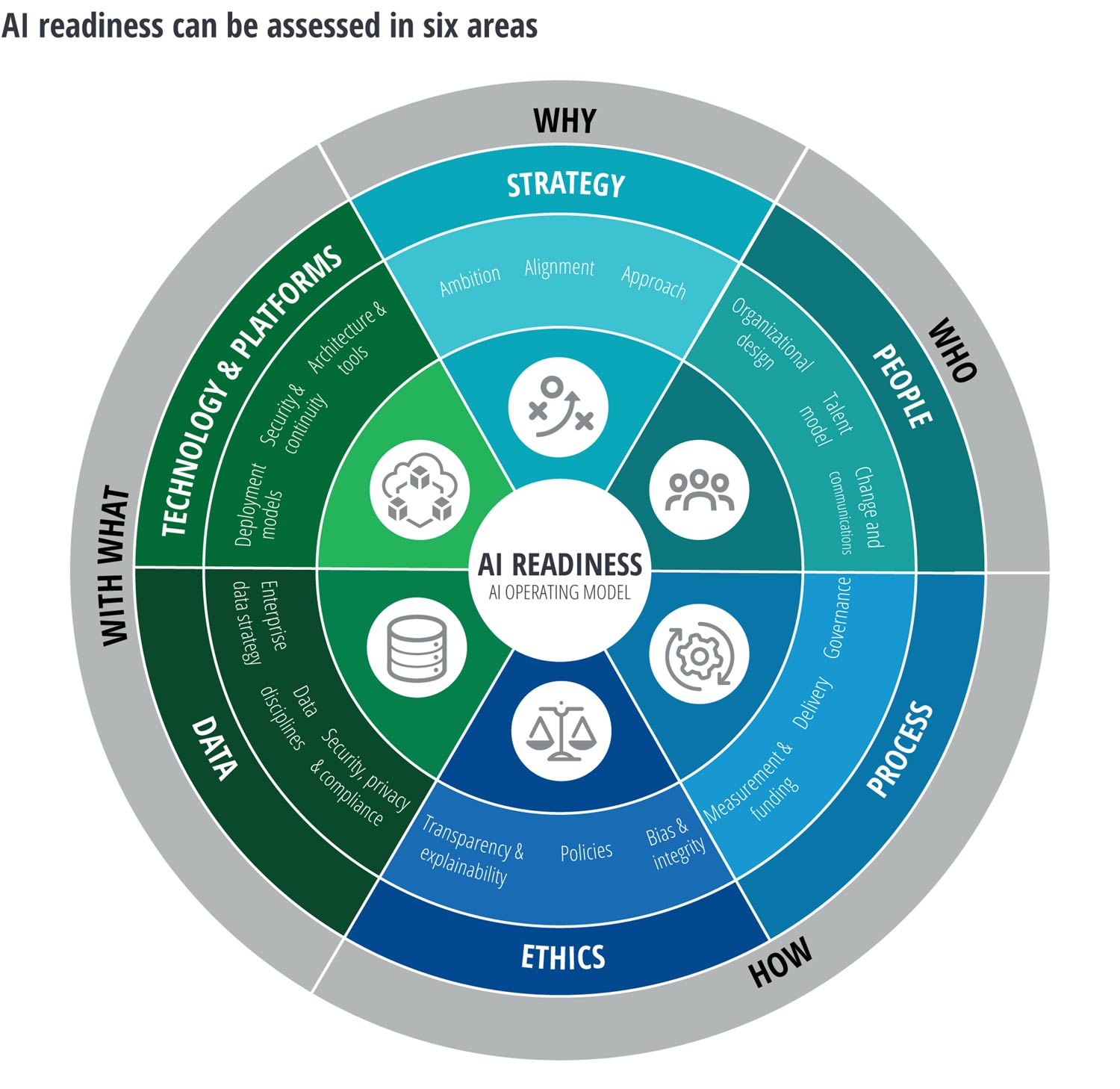
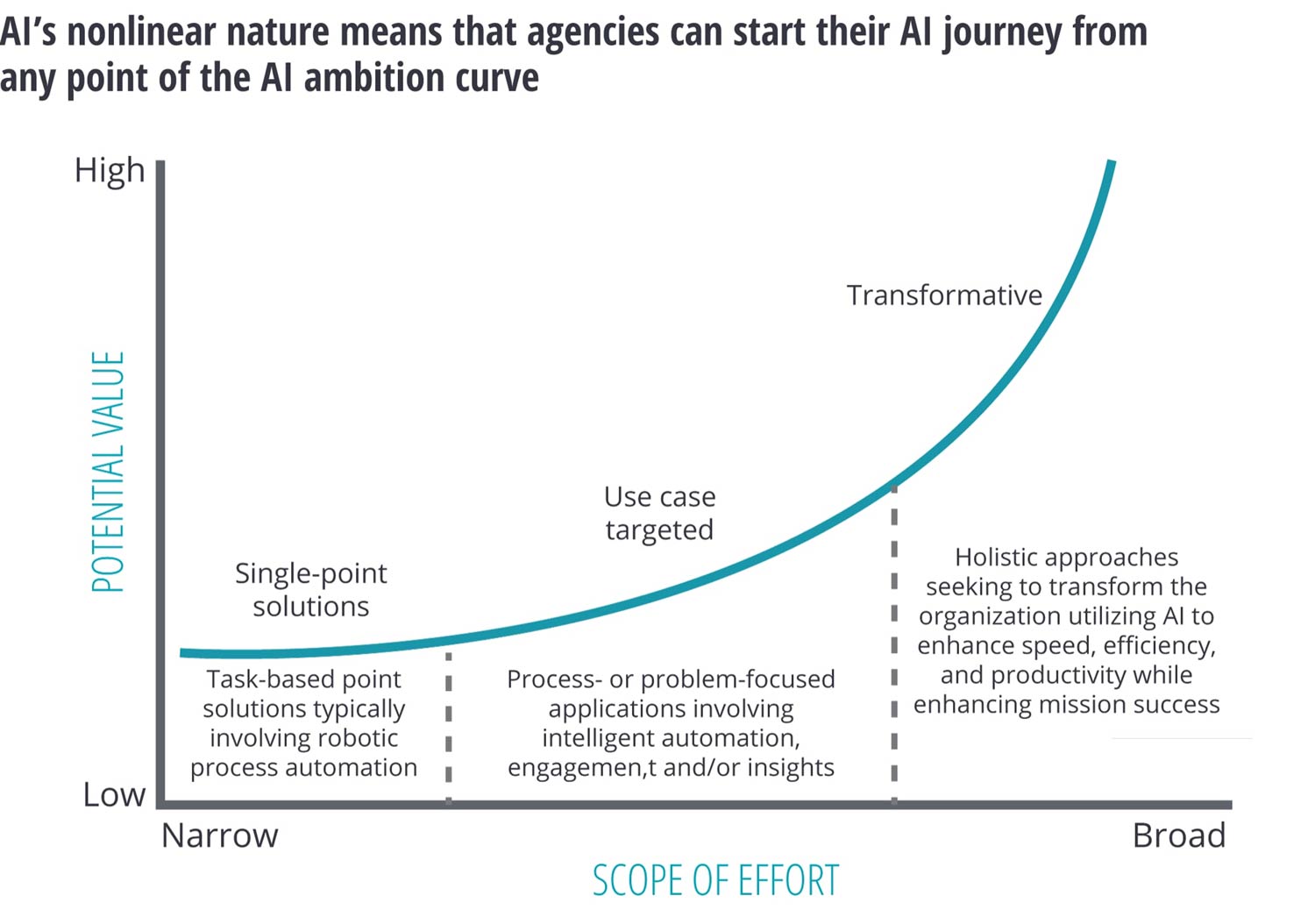
According to Deloitte’s AI readiness framework, all six areas mentioned should be considered in AI initiatives. However, the level of effort required depends on the organisation’s current state and the ambition for AI adoption. Ambition levels can be classified as:
- Narrow, single-point solutions;
- Process- or problem-focused use cases; and
- AI-fuelled transformation with the potential for the highest value and significant change.
Narrow solutions demand less effort, automating simple processes in standardised back-office areas, freeing up staff for more meaningful tasks and generating quick efficiencies. When multiple opportunities such as these exist, they can collectively deliver significant value.
Without an AI readiness assessment, an organisation can end up doing proofs of concept and other initiatives without understanding why they’re failing. Assessing readiness, meanwhile, allows an organisation to identify any gaps in its capabilities and address them before implementing an AI strategy.
- The author, Prof Mark Nasila, is FNB chief risk office chief data and analytics officer
- Read more articles by Mark Nasila on TechCentral
- This promoted content was paid for by the party concerned