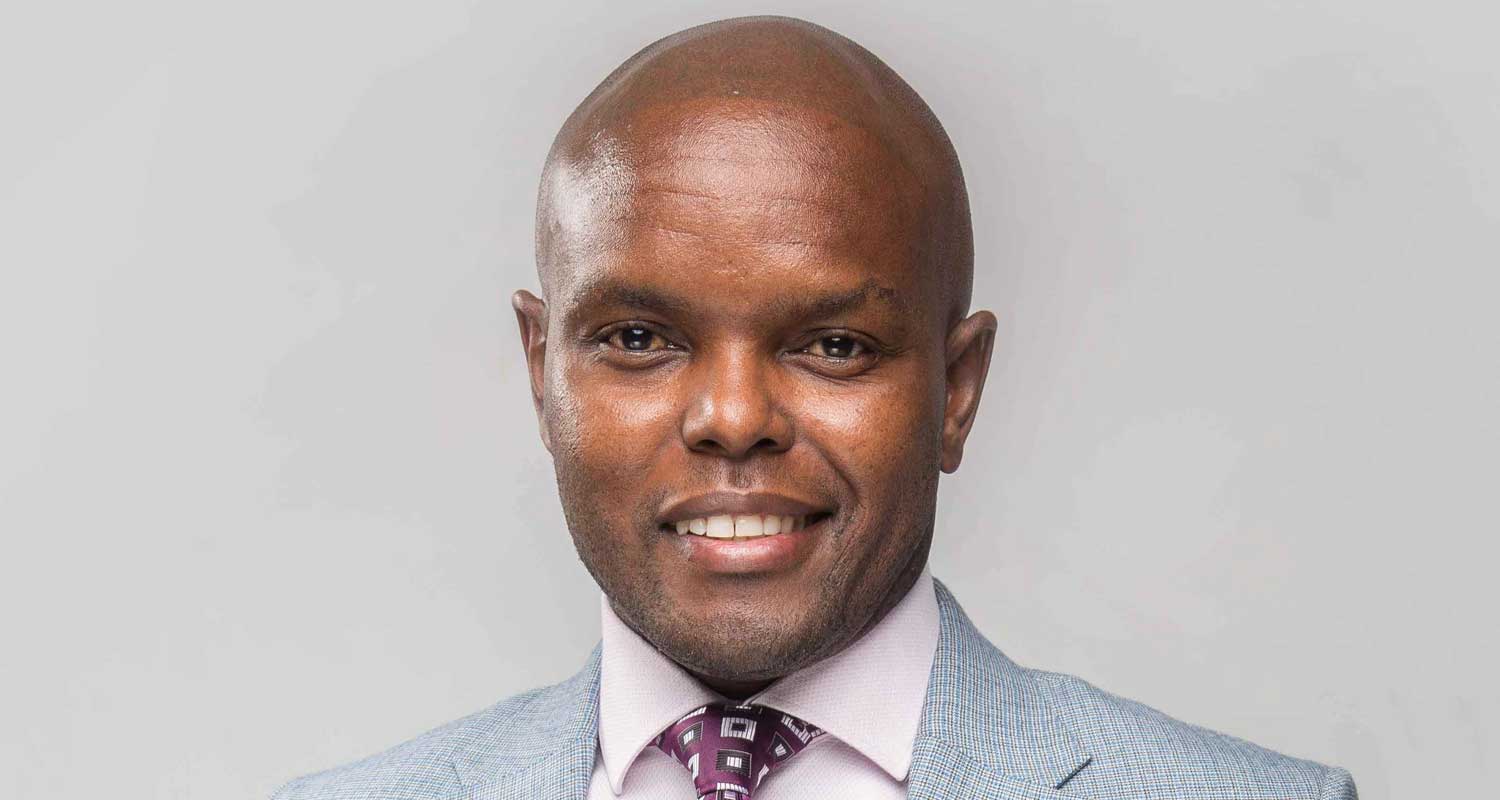
All artificial intelligence projects are driven by people — from conception to design, implementation and maintenance. As such, AI initiatives require the right people, with the right skill sets and enough capacity to dedicate sufficient energy to them if they’re to succeed.
Certain key roles are required: data scientists (who develop AI models), data engineers (who manage data sources and infrastructure), business analysts and, as projects grow, support from machine learning experts, DevOps engineers, AI strategists, data analysts, data architects and information governance specialists.
Outside of the FAANGs (Facebook, Amazon, Apple, Netflix and Google), few companies are fully equipped to successfully roll out AI initiatives, and finding the right talent comes with challenges of its own. For a start, there’s a scarcity of talent and huge competition for it. Then there’s the fact that experts in various AI-related fields are often reluctant to work for newcomers, making it hard for those businesses only just beginning their AI journeys to attract top talent.
Key roles for AI success
To return to the key roles above, one of the most common starting points for organisations looking to embrace AI is the hiring of a data scientist. Data science concerns data mining, big data and machine learning, and practitioners come in various forms and from varying educational backgrounds. Many study computer science, mathematics, statistics, engineering, physics or other quantitative fields
Ideally, a team will consist of a mixture of those with computer sciences, mathematics, statistics and business backgrounds. In the field of data science, there is a lot of overlap between job roles, with some roles focused on data analysis while others are more focused on applying insights to business decisions.
Less often appreciated or prioritised is the AI strategist, or as McKinsey refers to the role, an “analytics translator”. An AI strategist is responsible for converting business vision and goals into data and AI requirements, supervising project implementation, and ensuring that project results are integrated into business processes. This role is essential for successful data and AI projects since it bridges the gap between business and data science teams.
Data engineers are responsible for building and testing scalable big data ecosystems for businesses. They ensure that data systems are stable, highly optimised, and up to date with the latest technologies, enabling data scientists to run algorithms efficiently. Data engineers are also involved in the design and architecture of data engineering solutions. Their responsibilities include data replication, extraction, loading, cleansing and curating to ensure that data is ready for downstream reporting.
Collaboration
Machine learning engineers collaborate with data scientists and analysts to design and implement scalable and reliable data pipelines and services. Their work requires a deep understanding of AI and database technologies and involves performing A/B testing and implementing common machine learning algorithms such as classification and clustering. The ultimate goal of a machine learning engineer is to create self-running artificial intelligence to automate predictive models.
Other important roles include quantitative analysts, business intelligence analysts, data architects, data modellers, statisticians, business analysts, and the growing field of data ethicists — something getting ever more attention as the topic of ensuring responsible AI grows in importance.
A data ethicist is responsible for ensuring that data is collected, used and shared ethically and responsibly. Tasks include developing and enforcing data ethics policies, advising on ethical implications, addressing ethical issues, educating stakeholders on best practices, and working with data scientists. This field is growing and may require expertise in philosophy, law and computer science.
Just as important is the chief data and analytics officer (CDAO), a senior executive who leads an organisation’s data and analytics function, developing and implementing a strategy that aligns with the business’s goals and objectives.
The emerging spectrum of data and analytics roles
Gartner has delved deep into classifying various functions surrounding data, analytics and AI, and came up with four categories of roles: data and analytics roles, business roles, emerging roles and must-have roles. For example, under the last of these, you’ll find the CDAO, data translator, data engineer and data ethicist.
A solid foundation for a company looking to embrace AI would be to recruit a chief data and AI officer with expertise in business, data science and AI technology, with the skills to hire talent and establish teams. In addition to technical knowledge, strong leadership and communication skills are essential, as they will need to effectively communicate with individuals across various levels of the organisation.
It is recommended that companies build AI centres of excellence, with a diverse set of skills, including AI and IT professionals, business executives and domain experts, to bridge the gap between executive decision-making and AI implementation. A unified vision for AI across the enterprise needs to be created by standardising common practices and facilitating communication. 37% of the large companies in the US have already established such business units.
AI literacy across the organisation is also key. For example, to ensure that professionals are equipped to use AI tools effectively to improve outcomes, a four-step approach is suggested. This involves building awareness and capacity, enabling individuals to innovate and adopt new technologies, creating strategic partnerships, and developing knowledge exchange initiatives. The goal is to create an AI-enabled learning organisation that incorporates AI education and capacity building into its learning process.
As T. Fountaine, B. McCarthy, and T. Saleh argue in their article “Building the AI-powered organisation” in The Harvard Business Review: “Organisations must shed the mindset that an idea needs to be fully baked or a business tool must have every bell and whistle before it’s deployed. On the first iteration, AI applications rarely have the desired functionality. A test-and-learn mentality will reframe mistakes as a source of discoveries, reducing the fear of failure.”
The road to the successful implementation of AI can be a long one, but it starts with identifying the key roles for your particular use cases, identifying your existing resources — and gaps within them — deciding on the best approach to make up for any deficiencies, and, where it’s impossible to fill all roles rapidly, deciding which are the most important and starting there.
AI is only going to become more important for organisations, so knowing how to build effective AI teams won’t only serve you well for your first AI-powered project, but for those to follow.
- The author, Prof Mark Nasila, is chief data and analytics officer in FNB chief risk office
- Read more articles by Nasila on TechCentral
- This promoted content was paid for by the party concerned