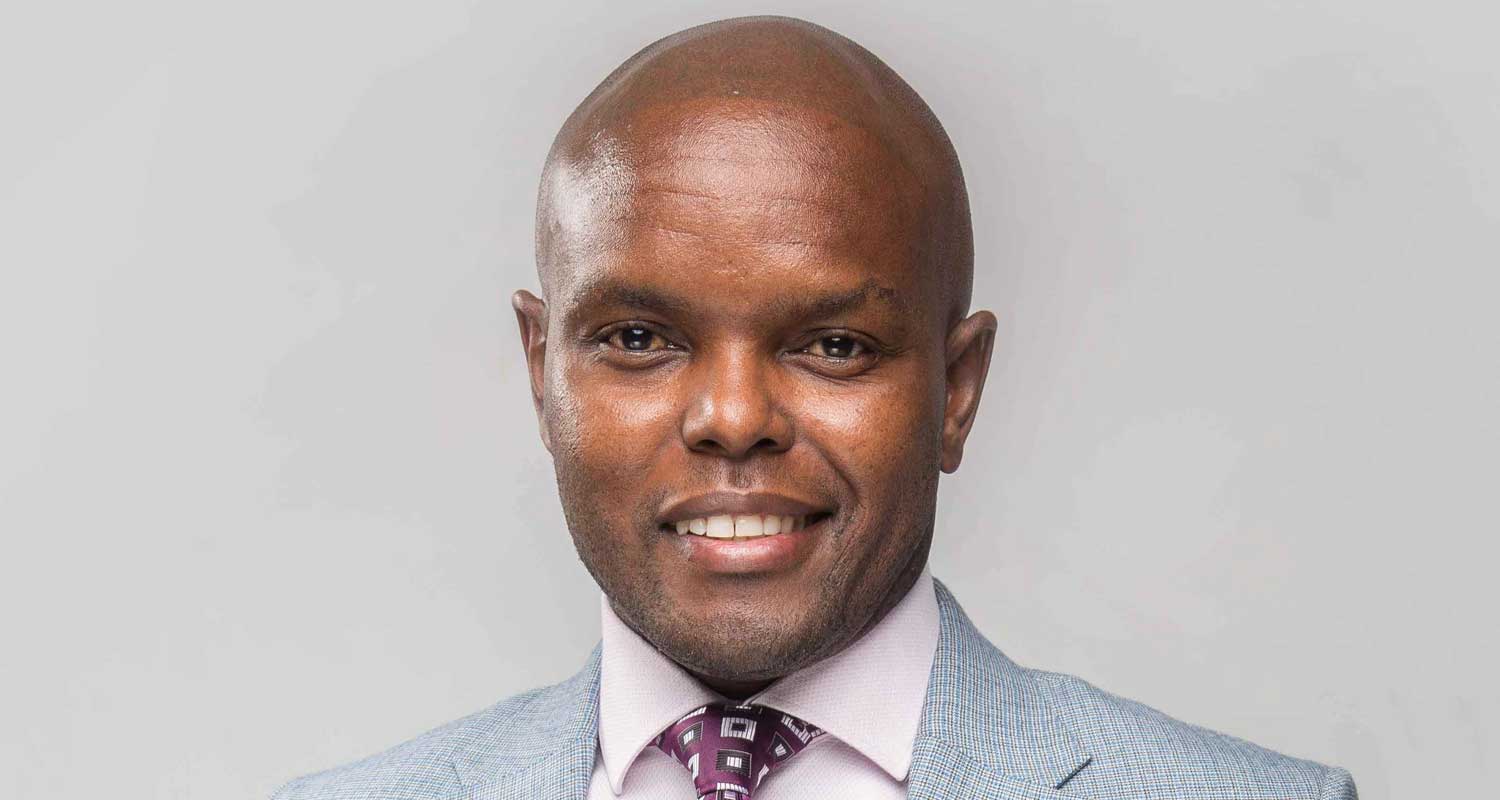
Every day, businesses have to make decisions – but decision making is complex, and getting it wrong can be costly. Decision intelligence (DI) is a relatively new field that harnesses data and elements of applied data science, social science and managerial science to help enterprises make better decisions. DI is also essential in implementing artificial intelligence (AI), and when combined with it, it’s a potent tool that can alleviate decision fatigue.
DI does this by linking data with decisions and outcomes and using various methods and tools, including decision mapping and decision theories, machine learning and automation. And there’s an obvious need for it. Forbes found there is a 95% correlation between decision effectiveness and financial performance, and according to McKinsey, bad decisions cost the average S&P 500 company $250-million a year.
First popularised by Lorien Pratt, Gartner identified DI as one of the world’s most impactful technology trends for 2022, and a growing number of businesses are embracing it. Cassie Kozyrkov, the chief decision scientist at Google, describes DI as “a new academic discipline concerned with all aspects of selecting between options,” and as “a unified field that helps people use data to improve their lives, their businesses and the world around them.”
DI is proving invaluable for the era of AI, because it helps people designing AI strategies and solutions to ensure they’re choosing the right sort of AI and applications for it, and that they’re responsibly creating appropriate metrics, objectives and safety nets, especially for automation at scale.
There are four key pillars underpinning DI. The first is managerial science, which is the interdisciplinary study of decision-making and problem-solving in organisations. The second, decision theory (sometimes called decision science), is the study of the logic and mathematics of decision-making under uncertainty, and how to make the best decision with the information available.
The third pillar is social science, which investigates human behaviour and includes disciplines like anthropology, sociology, political science and psychology. The fourth is data science, which concerns extracting useful information from data, along with adjacent fields like machine learning and big data.
Why is DI important for AI-focused companies?
DI is a commercial application of AI, focusing on the decision-making process. It’s always outcomes-focused and provides recommended actions that address specific business needs. A DI solution must deliver a return on investment by definition. Considering only 10% of AI models built by businesses are actually productised, there’s a massive opportunity for DI to improve that figure.
DI isn’t only about increasing implementation: it’s about improving outcomes.
“Is decision intelligence the new AI?” asks Pascal Bornet, AI expert and member of the Forbes Technology Council. Rather than DI replacing AI, what Bornet means is that DI can help organisations realise and unlock the possibilities of AI. By focusing on AI alone, many businesses aren’t realising the potential value of it, Bornet argues, but with DI, they can.
As Richard Potter, co-founder and CEO of DI services business Peak says, “artificial intelligence has a new confidant: decision intelligence”.
DI can augment decision-making by, for instance, helping humans decide between a huge array of options or complex data sets. Ultimately, the person still makes the final decision, but DI can help by simplifying the available options or rapidly weighing them against one another. When it comes to automation of the decision process, DI can empower AI to make decisions automatically based on existing rule sets and AI-based predictions.
DI isn’t about making human decision-making obsolete, though. It’s about changing their focus and making human decision-making better by empowering them with AI and a birds-eye view of their business so they can make the best decision given the information available to them at a particular time. It’s also about creating systems that learn from human feedback on previous decisions and incorporating that feedback into future decision-making.
The benefits are myriad, but some of the key ones are receiving actual, data-driven decisions from systems that can analyse past decisions and learn from them. While 91% of companies believe that data-driven decision-making can boost their business growth, only 57% of them rely on their data.
Another benefit is faster decisions. According to the McKinsey survey, only 20% of organisations are happy with their decision-making speed. DI also allows for multiple problem-solving options, allowing for flexibility when the parameters of a decision change, or predicting various outcomes based on different parameters. And — when appropriately trained and implemented — DI can help reduce mistakes and eliminate biases.
How businesses are using DI
Morgan Stanley’s wealth management platform uses DI to help customers reach their investment goals. Lloyds Banking Group, meanwhile, uses AI decision-making solutions across most of its business processes, from customising services to personalised customer communications.
In the retail sector, DI presents the opportunity to better predict product prices using information on demand, trends and even global macroeconomic variables. For example, software Remi AI lets users adjust their pricing to match customer expectations while also optimising their supply chains.
Enlitic Cure is a DI platform that combines AI and data analysis to help doctors prioritise cases speedily, analyse medical imaging reports, suggest diagnoses, and generally perform their jobs better, providing better care in the process.
Meanwhile, in the energy sector, tools like Athena AI helps energy producers automate decisions and reduce costs. It even predicts solar energy generation and optimises storage capacity in batteries accordingly. Infopulse is another energy sector DI tool used by Swedish utility Ellevio that enables it to pull data from multiple sources and use it to inform decision making.
It’s little wonder that DI is being adopted by companies like Google, Alibaba and Unilever. Google’s even gone as far as creating a DI division
One concern is a DI tool that can assist with ecological challenges too, from individual businesses to those facing society more broadly. One of the key benefits of decision intelligence is its ability to both harness massive historical datasets and also provide predictions that businesses and governments can use to prepare for potential challenges, like extreme weather. Moreover, DI can also help with creating the right strategies to contend with those challenges.
Texas-based Sabre Airline Solutions uses DI to optimise its booking and airline services solutions. The company also used DI to develop an “enterprise travel data warehouse” which enables it to monitor every aspect of its business around the clock, aggregating data from multiple services and using it to improve service levels and adapt its offerings to shifting customer needs.
DI integrates design thinking
A key component of successful DI is that it incorporates design thinking (DT). Under a design-thinking framework, a business needs to envisage what a customer wants and how a given decision will affect that same customer. Human-centred design — which starts with the customer’s needs and works backwards from them — can transform the way businesses develop AI products or services, but also how they make decisions.
Perhaps, for instance, historical data doesn’t match contemporary customer desires or needs. Because DT is iterative and constantly assesses whether solutions are customer-centric, it can flag the need to gather fresh data or consider alternative approaches.
Traditionally, DT has relied on qualitative research and metrics. But with DI, it’s now possible to use quantitative research and metrics for DT, too. That combination can lead to even better outcomes for customers and businesses alike.
It’s little wonder that DI is being adopted by companies like Google, Alibaba and Unilever. Google’s even gone as far as creating a DI division to help address the biases common to today’s AI systems. Alibaba has created its own Decision Intelligence Lab to improve efficiencies and reduce costs in everything from recommendations to analytics, and capital arrangement to the allocation of assets.
Unilever, meanwhile, is using DI to improve its supply chain by enabling faster decision-making. It’s also using AI to create new data-related careers that go beyond data entry. Reckitt Benckiser also uses DI to improve its supply chain, including supply chain forecasting.
DI may be a new discipline, but it’s one that’s here to stay; and it’s one that has the potential not only to help businesses realise the true potential of AI, but also to help them meet the actual needs of their customers today and into the future.
- The author, Prof Mark Nasila, is chief data and analytics officer in FNB’s chief risk office
- Read more articles by Mark Nasila on TechCentral
- This promoted content was paid for by the party concerned